The Intersection of AI and precision medicine at RSNA 2018
Images
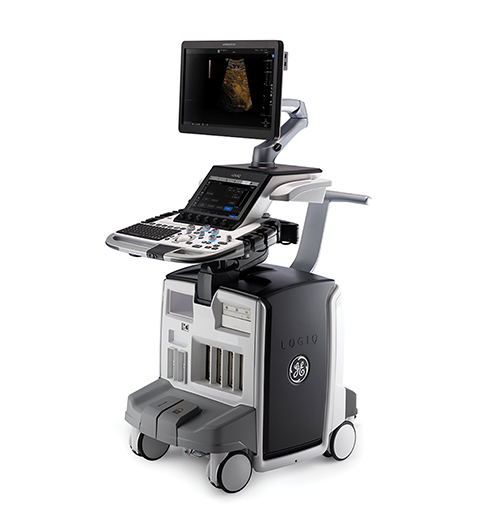
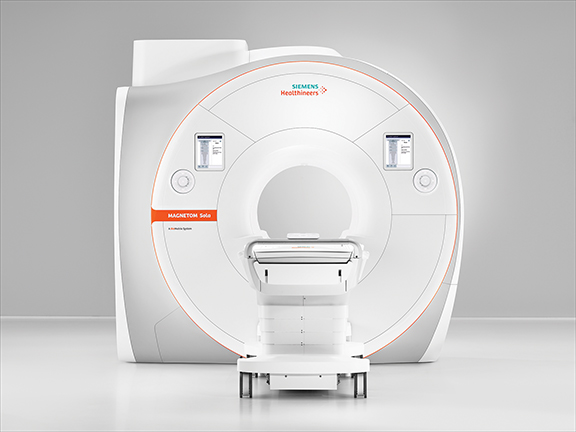
It’s a pretty safe bet that artificial intelligence (AI) will be the hot topic at RSNA again this year. We can expect to see a lot of interesting solutions, many that will likely be works-in-progress but also real products, too.
AI in radiology is in its infancy, says Michael P Recht, MD, Louis Marx Professor and Chair of the Department of Radiology at NYU Langone Health in New York City. Dr. Recht will present this year’s Annual Oration in Diagnostic Radiology at RSNA 2018; he will discuss how AI and machine learning will allow radiologists to become more integrated into the clinical care team.
“AI will completely change how we practice, from ordering exams and checking the appropriateness of an exam to how information is presented on the workstation for us to read and report. It will help us quantify data and make diagnoses and will overall make us better radiologists,” Dr. Recht says.
NYU Langone’s radiology department and Facebook announced a collaborative research project in August 2018 that will investigate using AI to make MRI scans up to 10 times faster. The project, fastMRI, will initially use 30,000 anonymized clinical cases consisting of greater than 10 million MR images of the knee, brain, and liver collected by NYU School of Medicine.
According to a Facebook post announcing the project, it may be possible to capture less data using AI, resulting in shorter scans, as well as potentially enhancing the rich information content of MR images. Artificial neural networks will be trained to recognize the underlying image structure and fill in the view omitted from the accelerated scan. These images will then be compared to traditional image reconstructions.
As Dr. Recht explains, medical images can be compressed, which removes data without impacting the image appearance. “The question is, if we can remove data, why not just under sample and then create the image that we want,” says Dr. Recht. “In MR we can save time; in CT we can reduce radiation exposure.”
Working with the Facebook Artificial Intelligence Research (FAIR) group, which was looking for projects where AI could have a real-world impact, brings together data scientists and academic imaging scientists and radiologists. Dr. Recht believes it is paramount that radiology partners with organizations that have expertise in AI and data sciences, and he’s excited at the potential opportunities of this collaboration.
“It’s a challenging problem, but think about the potential,” Dr. Recht adds. “This project could have a significant impact helping a large number of people throughout the world, whether it is in underdeveloped regions where there are only one or two MRI systems or in countries where there are large backlogs for MRI exams. If we can make MR faster, then we can perform more scans and help more people.”
The fastMRI project signifies a different approach to bringing AI into radiology in addition to pattern recognition for image analysis. AI in health care shouldn’t be focused only on pattern recognition, says Gavin Lew, managing director at Bold Insight, a full service user experience research agency with expertise in the medical device and digital health industries.
“If all we do is have AI mimic humans then we lack context and are doing radiology a disservice,” Lew says. “AI is really good at identifying interesting correlations. Why not have AI output as one voice into a system with clinicians, researchers and others, so we are not just replicating but actually helping humans make better decisions?”
For example, AI can help aggregate data from the patient’s chart and make it available to the radiologist while reading an imaging study. It can deliver more insight into a diagnosis through meta-analysis of the patient’s history, genetics and co-morbidities. It’s using AI to provide a higher level of decision support so that the radiologist can then apply their clinical expertise to deliver more insight into the diagnosis. That’s the interaction and context that can build trust and set higher goals for AI in radiology, Lew says.
Researchers and industry also need to take the time to understand the data sets. It’s not just throwing in the data to feed the algorithm because we can, Lew explains. Rather it is how that data output will generate human interaction that is important.
“A computer will identify correlations or patterns and then we need the doctor or researchers to look at those differences and determine how we can improve diagnoses or therapies through those differences,” Lew says. “If the holy grail is better medicine and better health, then how can AI act as an assisted clinical system? That’s where the focus should be—the differentials are more interesting than the correlations and that is what clinicians and scientists should further investigate.”
That view of assisted intelligence rather than artificial intelligence is one that Dr. Recht shares.
“I see AI and machine learning integrating into almost every aspect of radiology, assisting us and making us more accurate,” Dr. Recht says, adding that for the past 20 years, radiologists have become more efficient and productive, but AI has the potential to make radiologists more accurate—which is a key component of precision medicine and personalized health care.
It’s not just imaging data and radiomics, but genetics and pathology where machine learning is needed to help radiologists access, understand, and apply all the patient data available to them.
“When I started in the field, radiology was at the center of medical care,” Dr. Recht explains. “If there was a problem, the doctor went down to radiology for help solving it. Today, we are pattern recognizers. We need to go back to understanding the clinical context and then interpreting the images so we can deliver a better analysis of the patient’s situation—whether that situation involves disease or injury. With AI, we can do this quicker and better, and radiologists can go back to being more integrated into the clinical team. AI can help to make us a more valuable consultant and offer more value to patients.”
AI-infused solutions at RSNA
At RSNA 2018, Canon Medical Systems will focus largely on AI, beginning with introducing Collaborative Imaging. Collaborative Imaging puts technologies together, using multiple modalities and innovative clinical applications to deliver embedded, integrated applications in each step of the care pathway, from acquisition to post processing.
“Our new offering is a new way of thinking,” says Satrajit Misra, vice president, Marketing and Strategic Development, for Canon Medical Systems USA. “It isn’t centered on the patient’s needs today or even tomorrow. It’s a holistic approach to their care throughout their entire treatment lifecycle, which could be days, months or years.”
It’s about thinking bigger than what’s directly in front of us, Misra adds, and forging new ground for the diagnostic imaging industry that may lead to significant improvements in patient care.
Improving clinical, operational and financial outcomes through better workflows and smarter use of data is a primary focus for Change Healthcare. At RSNA, the company will demonstrate how its workflow orchestration solution drives smarter study prioritization and additional workflows using AI as well as provide assistive diagnostic tools for the physicians. The company will also highlight work in areas that enable more efficient, accurate, and safer imaging.
“We believe that AI will have a significant role in enabling better outcome, and we are advancing our AI offering through both organic research and development, partners integration and customers collaborations,” says Philippe Raffy, PhD, executive director of artificial intelligence at Change Healthcare.
At last year’s RSNA, GE Healthcare and NVIDIA announced a deepening of their 10-year partnership to enable advanced AI applications and accelerate the speed at which medical imaging data can be processed. Across its entire imaging portfolio, the company says it has 200-plus imaging apps and, in addition to NVIDIA, is working with Microsoft, Amazon and Intel to embed analytics, cloud capability, and AI into devices and workflows. GE is also working with other medical industry innovators to address hospitals’ and patients’ needs across the entire care pathway.
“Take, for instance, our recent collaboration with Roche Diagnostics, which is focused initially on acute care and oncology,” says Tom McGuinness, president and CEO of GE Healthcare Imaging. “This partnership combines in vivo data from GE Healthcare’s advanced analytics, medical imaging and monitoring equipment with the in vitro data from Roche’s biomarker, tissue pathology, genomics and sequencing portfolio.”
GE’s new Revolution Frontier CT uses NVIDIA’s AI computing platform to process images two-times faster than its predecessor. The company says this speed helps with liver lesion detection and kidney lesion characterization and could potentially reduce the need for unnecessary follow-ups and non-interpretable scans when Gemstone Spectral Imaging Metal Artifact Reduction software is utilized.
In ultrasound, LOGIQ E10 acquires data in a similar way to an MRI or CT system and then leverages advanced GPU hardware technology that the company says delivers 48 times the data throughput and 10 times the processing power of previous systems to reconstruct the ultrasound images in real-time. The same NVIDIA architecture being used to power driverless cars and the next generation of 3D video gaming underlies the ultrasound system’s platform, cSound Architecture.
GE will also highlight free-breathing scan capabilities on ViosWorks, a cardiac MRI solution that can reduce the traditional lengthy cardiac MR exam to less than 10 minutes. In addition, the company says, ViosWorks features industry-first clinical deep learning applications aimed at one-click cardiac MRI exams, which can analyze incredibly large datasets and help support quicker treatment decisions for patients.
Supported by the Lumada platform services, Hitachi Healthcare Americas Corporation will preview a works-in-progress AI imaging solution that supports scanning, diagnosis and reporting to improve the quality of daily examinations. Key features include supporting scan positioning, setting the slice position, reducing scan times and improving quantification. The company says that this functionality will help radiology become more efficient and effective in reporting, allowing for the increasing volumes of patients to be more easily handled.
Early this year, Hitachi acquired VidiStar, LLC, to further advance the company’s informatics strategy and analytics capabilities to improve workflow and intelligence for physicians, particularly in diagnostic ultrasound examinations. VidiStar provides a structured reporting and image management system that enables analysis of patient data to help physicians make decisions. Hitachi will be demonstrating its progress in analysis and reporting through digital technologies that are enabled by diagnostic imaging with AI.
Informatics with AI is a key focus for Philips Healthcare. However, Homer Pien, PhD, Chief Scientific Officer, refers to AI as adaptive intelligence. “It is matching minds and machines to sharpen intelligence and insights,” Dr. Pien says, adding that adaptive intelligence can provide the necessary contextual understanding of features that are important and relevant to the task at hand.
“The difference between correlation and causation is really important,” Dr. Pien explains. “Human beings were trained to understand confounding factors, but it’s hard for an AI algorithm to do that, and even harder to train that data properly.”
AI is needed to help clinicians make sense of all the data, Pien adds. Philips is focused on building an integrated diagnosis with the information that resides in patient data. Illumeo, Philips’ enterprise imaging PACS, is designed to empower the radiologist with knowledge. In addition, the company’s IntelliSpace advanced visualization platform aggregates information for the clinician. The company is focused on enhancing the solution so that it is more predictive for the future.
Philips’ recent agreement with Dana-Farber Cancer Institute that will deploy Dana-Farber Clinical Pathways into the company’s IntelliSpace Oncology Platform is an example of providing information and intelligence that can help clinicians through the use of decision trees.
“Clinicians can make intelligent choices by looking at real outcomes data to see if one treatment path has a better outcome than another,” Dr. Pien says. “We’re not there yet, but the Dana-Farber Clinical Pathway is a very significant first step.”
According to Joerg Aumueller, global head of digitalizing healthcare marketing at Siemens Healthineers, the company has over 500 patents on machine learning, more than 100 patents in deep learning, and over 40 AI-enriched offerings in its product portfolios.
For example, AI can be used to help find landmarks in the body to enhance accuracy in image acquisition and also improve workflow. The company will showcase its recently FDA-cleared MAGNETOM Sola, a 1.5T MRI system that utilizes AI and body models to expedite patient positioning and deliver consistent, reproducible results.
In CT, the use of deep learning algorithms can automatically adjust patient positioning, find the best isocenter for the patient as well as provide information on the optimal scan range and directions. In radiology interpretations, AI can improve workflow and efficiency by delivering reading assistance to help identify and highlight abnormalities in X-ray and CT images. Another example is the auto-contouring of organs at risk, a time-consuming task, in Siemen’s radiation therapy suite to improve workflow.
“AI is specific to productivity and efficiency gains to transform care delivery. On the other hand it could turn out to expand precision medicine as well: the ability to increase accuracy using the same amount of time,” Aumueller says.
“Combining different data at the point of decision making can help radiologists deliver a more precise diagnosis,” Aumueller adds. “We need to generate data at the point of acquisition to start with a more accurate beginning and then aggregate that data with other sources to finally analyze it with the help of technologies like AI turning data into insights. The challenge is how to move from unstructured to structured data that is relevant and accessible.”
Canon Medical believes the cornerstone of precision health is imaging intelligence. “That’s where collaborative imaging comes in, as it provides clinical applications across disciplines and modalities—delivering focused, personalized patient centric care during every step of the journey,” says Misra. “It puts embedded, integrated applications at the center of patient centric care from scanning, to diagnosing, to sharing and analyzing.”
A purposeful blend of precision diagnostics, therapeutics, and monitoring tailored to each patient is what GE calls precision health. “Precision health will help deliver health care’s triple aim: better quality care at a lower cost with increased access for patients around the world,” explains McGuinness. “We envision a future where data, analytics and individualized care help accurately diagnose disease, comprehensively monitor a treatment’s effectiveness and improve the patient experience.”
Citation
Massat MB. The Intersection of AI and precision medicine at RSNA 2018. Appl Radiol. 2018;(11):24-27.
November 8, 2018