Demystifying AI: Going beyond escape velocity
Images
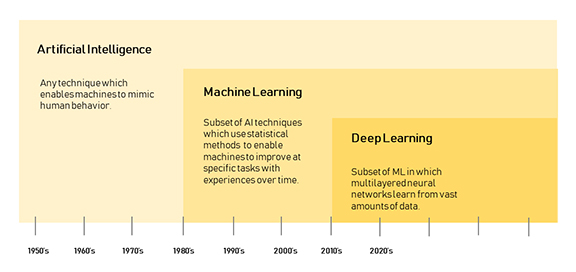
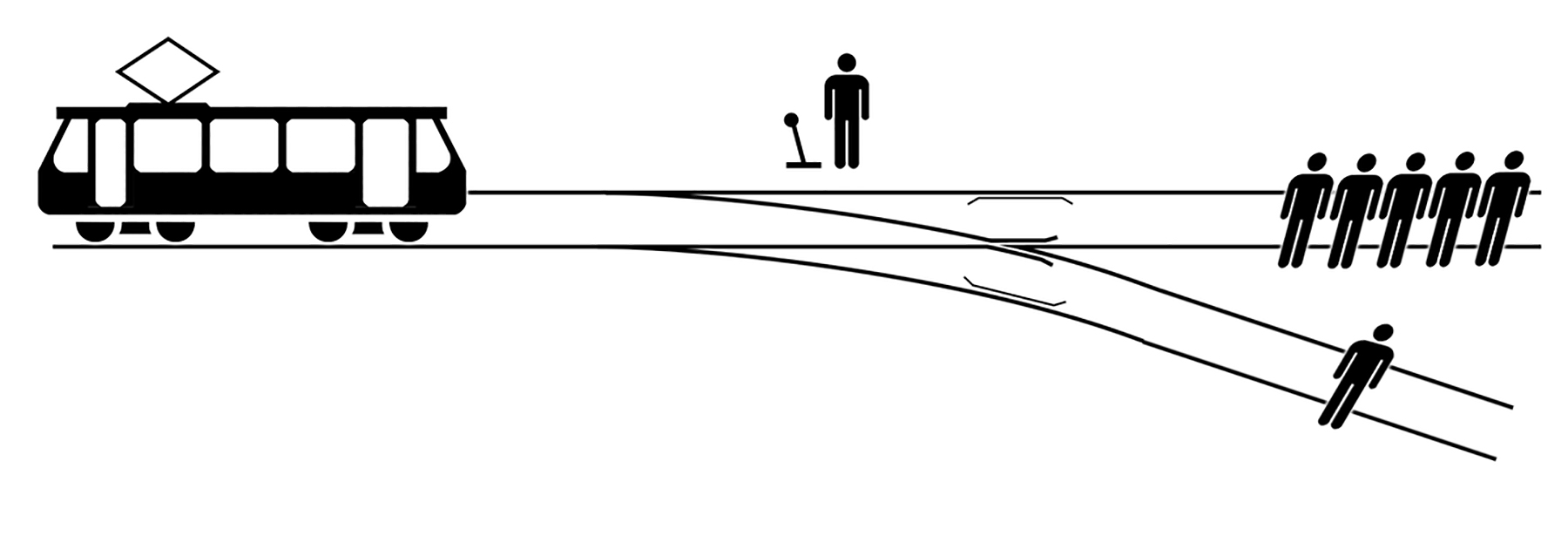
“By far, the greatest danger of artificial intelligence is that people conclude too early that they understand it.”
—Eliezer Yudkowsky
In the world of failing attention spans and rising opportunity costs, the hype cycle of artificial intelligence (AI) is seemingly at an all-time high.
In physics, escape velocity is the minimum speed needed for any object to escape the gravitational influence of a massive body, such as a planet or moon. We’ve seen this phenomenon in those spectacular space shuttle launches. And we may be about to witness it in the brave new world where artificial intelligence will soon be a natural phenomenon.
Rhetoric vs reason
Interest, influence, and investment in AI have skyrocketed over the past several years to a point where if you’re not “doing AI,” then you’re not doing anything right. One recent survey found that up to 80 percent of participating enterprises have some form of AI in production today, with another 30 percent planning to expand AI investment over the next three years. It gets even more interesting in health care where globally, 63 percent of healthcare executives say they already actively invest in AI technologies, and 74 percent say they are planning to do so.1 By 2025, 90 percent of U.S. hospitals will use AI to save lives and improve quality of care.2
The airwaves are filled with data points and bold statements that challenge reason with rhetoric. Innovator and entrepreneur Elon Musk has warned that AI presents a “fundamental existential risk for human civilization,” and that the global race for AI supremacy may be the most likely cause of a third world war. Not long ago, China announced its “New Generation Artificial Intelligence Development Plan.”3 Andrew Ng, former Baidu Chief Scientist, Coursera co-founder and Stanford professor, insists that AI is bigger than electricity.4
There’s good reason to steer through the rhetoric and attempt to find our “escape velocity.” We in healthcare innovation are especially aware of the real potentials for AI: Technology that could swiftly review hundreds of thousands of medical journals to ensure no newer, more suitable treatment path exists; algorithms that can predict risk for a heart attack and when; and solutions that can guide less-experienced clinicians in interpreting images, helping them to identify a diagnosis that may otherwise be missed.
Numbed by nomenclature
With so much potential for progress, how can we avoid being blinded by the buzzwords, and numbed by the nomenclature surrounding AI? The way we talk about AI, and indeed the very language we use to describe what it does, should be more measured, pragmatic, and clear. The language we use in conversations around AI must empower understanding so we can embrace what we support and challenge what we don’t. Clarity is king, and AI should leave no room for hyperbole, fear mongering, or jargon. TIME magazine’s recent Special Edition, “Artificial Intelligence, The Future of Humankind,” kicked off with a glossary of AI terms. (See Figure 1, which clarifies the terms most commonly used with respect to the evolution of artificial intelligence.)
Human interface: reinvent vs replace
As we push towards escape velocity in AI, let’s also take a lesson from healthcare’s past: The embrace of digital in the past three decades has merely been an attempt to replicate the analogue workflow and analogue culture in a digital form. Instead, we must reinvent the workflows so digital performs better than analogue ever did. For AI, I suggest we go beyond comparison to humans. AI isn’t a replacement of human capabilities. It’s a reinvention of what it means to leverage the power of machines at scale, and a way to augment the most humanistic aspects of care.
Between 2010 and 2015, the amount of stored patient data increased 700 percent; the vast majority of this data is unstructured data. Targeted use of technologies to harness the potential of that data could make patient care more efficient and cost effective.
With demand for medical imaging and specialized diagnostics still on the rise, many countries struggle to meet the need for radiologists. Consequently, many radiologists are working at full throttle – not just with increasing work volumes, but under mounting pressure due to declining reimbursement rates and the transition to value-based care models. Manual interpretation of medical images is often subjective, requiring a combination of experience and intuition, and can lead to a level of clinical hedging and clinical errors. In addition to a frightening increase in medical errors and heightened malpractice risk, medical burnout is also incredibly expensive. JAMA estimates a hospital with 450 doctors loses $5.6 million per year due to high physician burnout and turnover. This leads to increased costs, which can be calamitous for patients. The promise of AI is to augment the human interface, allowing doctors to be healers, not just computer data entry clerks. Radiologists, as we know them today in the volume-based, “digital 1.0” world, may indeed be replaced with radiologists of the “digital 2.0” world, where their role, augmented by AI, evolves from that of mere diagnostician to one of physician consultant.
AI plus ethics
While most discussion around AI focuses heavily on decision support – helping provide insights and helping to draw out conclusions---the next phase of AI will more intelligently automate action from data. This calls for a heightened sense of awareness and control to address real issues that may affect AI- based clinical care; these include bias, privacy, diversity, ethics, continuous model learning and trust. When AI-based cancer-spotting image recognition is trained only on fair-skinned people, bias becomes real, and trust and confidence in the data deteriorate rapidly. Data integrity is critical, especially when dealing with data at scale with the capabilities that AI brings to the table. Heightened caution is required around bias because AI often perpetuates existing biases based on generated learning models that deepen false assumptions. A popular saying is that natural stupidity is more dangerous than artificial intelligence. Our goal should be to enhance and augment intelligence.
The canary in the coal mine for health care as it relates to AI could be the automotive industry. Pittsburgh is the AI home base for at least four autonomous car companies. 5 The city also offers easier access to talent coming out of Carnegie Mellon University, home of the world’s first machine learning department, alongside a rich terrain that offers up challenges galore to sensors, software, and decision points. One might think that, with the likes of Tesla, Uber and Argo.ai heavily invested in self-driving vehicles, all activities would be on autopilot by now; however, it’s definitely stop-and-go traffic for the industry. Much of this, just as in health care, will boil down to balancing science, technology, and our readiness to embrace evolving ethics and rules with respect to how and why we use AI.
The “Trolley Dilemma”6 consists of a series of hypothetical scenarios developed in 1967 by British philosopher Philippa Foot (Figure 2). American philosopher Judith Jarvis Thomas further scrutinized and expanded on the idea in The Yale Law Journal. Here’s a rundown for those not familiar: a runaway trolley is rumbling down a track, with five workers ahead who are sure to be killed if the trolley reaches them. A lever can be thrown to switch the trolley to an adjacent track, but there’s a worker on that one as well who would likely be doomed. The question for the AI algorithm is: Does one throw the switch and kill one person, or does one do nothing and kill five? Data scientists in universities, autonomous car companies and, now, healthcare systems, often grapple with this age-old dilemma, which is not just a simple binary choice, but also a debate of conscience, emotion, perception, and perhaps even logic and law.
Earlier this year, I had the privilege to participate in a first of its kind “AI + Ethics” conference at Carnegie Mellon University,7 where experts from academia, industry, government, and the media came together and discussed hard questions beyond slogans and soundbites. Accountability, privacy, regulations and morality are key issues that need to be front and center in the pursuit of meaningful AI in health care.
AI Market vs AI marketing
There has been a 14-fold increase in the number of active AI startups since 2000. But when the marketing engine tries to sell things faster than the products can be put in the “back of the truck,” chaos ensues. The gravitational lure of an AI-driven marketplace has caused bumpy rides to many well-intentioned companies. A report issued in July by Jefferies LLC,8 argues that despite IBM’s heavy investment in Watson (which the analyst estimated at $15 billion from 2010 to 2015 alone), the division won’t be profitable. And just as this paper was going to press, news broke that Deborah DiSanzo, the division’s head, would be stepping down and moving to another team within the company.
A recent KLAS report, titled Artificial Intelligence in Imaging 2018,9 detailed the efforts of 81 healthcare organizations to pursue meaningful use of AI. The report noted that of all the areas with potential AI applications, medical imaging has received the greatest attention. Vendors and providers in this space are looking forward to using AI to improve diagnostic processes, develop treatment protocols, and personalize patient care, among other potential uses. Surprisingly, almost half of the organizations surveyed are already live with AI, or making plans to employ it in medical imaging. The report noted that many organizations use their AI tools in a limited way, typically within a single department (eg, radiology), as pilots to learn more about AI’s potential.
A plethora of activities are unfolding among vendors in the enterprise imaging space. GE Healthcare has built on a longstanding partnership with Nvidia to bring AI directly to imaging devices around the world. Cloud companies, such as Google Cloud, have made meaningful strides working with IT companies to create tools using AI in clinical workflows, analytics, and storage. Startups such as MD.ai and Zebra are approaching AI and algorithmic development with an unprecedented level of nimbleness.
And there’s more coming down the pipeline from leading entrepreneurial academic medical centers. Stanford’s Machine Learning group, led by Pranav Rajpurkar and others, including Andrew Ng, developed CheXNet,10 an algorithm that can detect pneumonia from chest X-rays at a level exceeding practicing radiologists. CheXNet is a 212-layer convolutional neutral network that inputs a chest X-ray image and outputs the probability of pneumonia along with a heat map localizing areas of the image most indicative of pneumonia. We’re going to be seeing more developments happening in this space, not just in deterministic AI algorithms, but also in probabilistic algorithms.
What’s mind-blowing is that in a recent hackathon I was judging at the University of Pittsburgh’s Innovation Institute,11 one of the winners was a team of three data science students from Carnegie Mellon University who, over one weekend, took the same datasets from the Stanford ML group and actually bettered the Stanford scores.
AI Reset
As AI veers towards escape velocity, a core ingredient that is in short supply consists of the right skills to to truly capitalize on these new innovations. We need more data scientists, and we need to make sure clinicians get formalized training in data science. The medical education curriculum needs a reset to accommodate this dire need. The health ailments of tomorrow will not just be fought with X-rays and stethoscopes – they will be cured with data and AI.
The AI frenzy appears to be triggering a rush to build anything with AI attached, including algorithms, apps, and point solutions. If data is king, and algorithms will soon be a “dime a dozen,” then perhaps we need to focus on the delivery mechanisms and the value of AI capabilities. Perhaps we need an “AI reset” and to match our goals and ambitions with capabilities and clinical and business imperatives.
The promise of AI is not waning; indeed, it is brighter than ever. What we need to do, however, is to become more purposeful, think more holistically, and use AI to actually humanize health care.
References
- Williams, Brian. Enabling Better Healthcare With Artificial Intelligence. s.l. : PWC, 2017.
- Das, Reenita. Five Technologies That Will Disrupt Healthcare By 2020. Forbes. [Online] March 30, 2016. [Cited: October 18, 2018.] https://www.forbes.com/sites/reenitadas/2016/03/30/top-5-technologies-disrupting-healthcare-by-2020/#19dd6f6b6826.
- Kania, Elsa. Emerging technology could make China the world’s next innovation superpower. The Hill. [Online] November 6, 2017. [Cited: October 20, 2018.] https://thehill.com/opinion/technology/358802-emerging-technology-could-make-china-the-worlds-next-innovation-superpower.
- Li, Oscar. Artificial Intelligence is the New Electricity — Andrew Ng. Medium. [Online] April 28, 2017. [Cited: October 23, 2018.] https://medium.com/syncedreview/artificial-intelligence-is-the-new-electricity-andrew-ng-cc132ea6264.
- Levy, Ari. Pittsburgh’s self-driving car boom means $200,000 pay packages for robotics grads. CNBC. [Online] September 16, 2017. [Cited: October 12, 2018.] https://www.cnbc.com/2017/09/16/pittsburghs-self-driving-car-boom-means-200000-pay-packages-for-robotics-grads.html.
- Trolley problem. Wikipedia. [Online] [Cited: October 18, 2018.] https://en.wikipedia.org/wiki/Trolley_problem.
- The Carnegie Mellon University - K&L Gates Conference on Ethics and AI. CMU. [Online] [Cited: October 16, 2018.] https://www.cmu.edu/ethics-ai/.
- Creating Shareholder Value with AI? Not so Elementary, My Dear Watson. Bluematrix. [Online] July 12, 2017. [Cited: October 18, 2018.] https://javatar.bluematrix.com/pdf/fO5xcWjc.
- KLAS. Artificial Intelligence in Imaging 2018. s.l. : KLAS Research, February 2018.
- Pranav Rajpurkar, Jeremy Irvin, Kaylie Zhu, Brandon Yang, Hershel Mehta, Tony Duan, Daisy Ding, Aarti Bagul, Curtis Langlotz, Katie Shpanskaya, Matthew P. Lungren, Andrew Y. Ng. CheXNet: Radiologist-Level Pneumonia Detection on Chest X-Rays with Deep Learning. [Online] December 25, 2017. https://arxiv.org/abs/1711.05225.
- Bluehack. University of Pittsburgh Innovation Institute. [Online] October 14, 2018. https://www.innovation.pitt.edu/events-competitions/bluehack/.
Related Articles
Citation
Shrestha RB. Demystifying AI: Going beyond escape velocity. Appl Radiol. 2018;(11):8-11.
November 9, 2018