AI Plus Volumetric Thresholds May Enable Large-Scale Splenomegaly Screening
Images
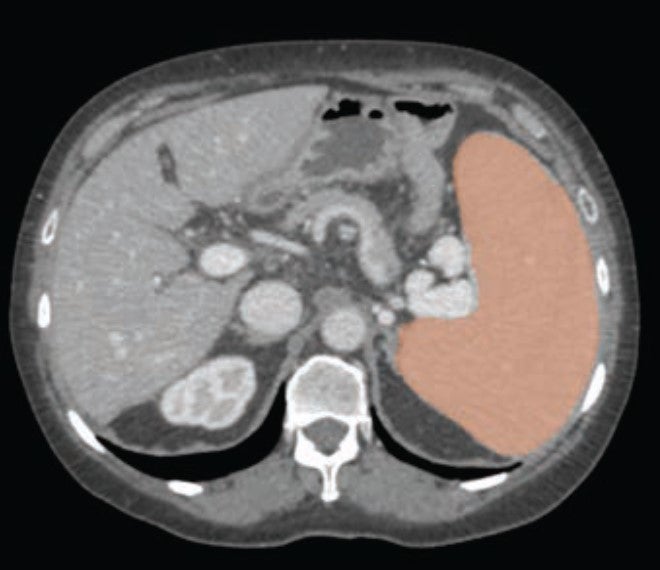
Using an automated deep-learning AI tool, as well as weight-based volumetric thresholds, might afford large-scale evaluation for splenomegaly on CT examinations performed for any indication, according to research published in the American Journal of Roentgenology.
Noting that, historically, the standard linear splenic measurements used as a surrogate for splenic volume yielded suboptimal performance in detecting volume-based splenomegaly, “the weight-based volumetric thresholds indicated the presence of splenomegaly in most patients who underwent pre-liver transplant CT,” explained corresponding author Perry J. Pickhardt, MD, from the department of radiology at University of Wisconsin School of Medicine & Public Health.
The study included a screening sample of 8,901 patients (4,235 men, 4,666 women; mean age, 56 years) who underwent CT colonoscopy (n = 7736) or renal-donor CT (n = 1165) from April 2004 to January 2017. A secondary cohort of 104 patients (62 men, 42 women; mean age, 56 years) with end-stage liver disease underwent pre-liver transplant CT from January 2011 to May 2013. Pickhardt et al.’s deep learning algorithm—previously developed, trained, and tested at the National Institutes of Health Clinical Center—was used for spleen segmentation, to help determine splenic volumes, with two radiologists independently reviewing a subset of said segmentations.
Ultimately, this automated deep-learning AI tool was utilized to calculate splenic volumes from CT examinations in 8,853 patients from the primary outpatient population. Additionally, splenic volume was most strongly associated with weight, among a range of patient factors.
“To our knowledge, this study represents the largest reported sample of patients to undergo volumetric segmentation of the spleen,” the authors concluded.