AI Models Seek to Make Brain MRI More Accurate and Reliable
Images
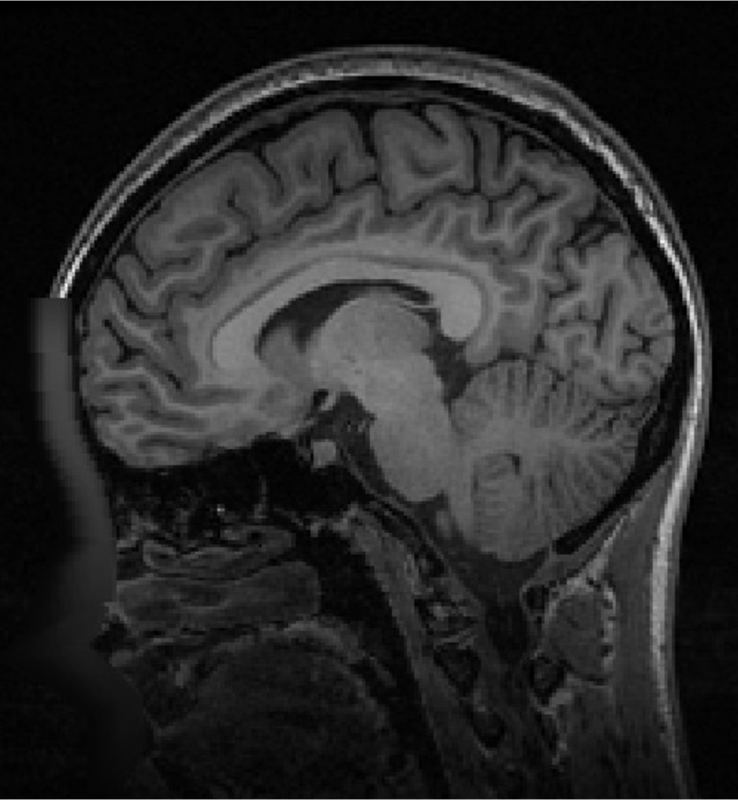
Researchers have created two new generative artificial intelligence (AI) models to help improve the image quality of brain MRI. One model can more accurately remove non-brain tissue from images and the other that can greatly enhance imaging quality. The recent papers from the lab of Li Wang, PhD, associate professor in the Department of Radiology at the University of North Carolina School of Medicine were both published in the journal Nature Biomedical Engineering.
“Imaging quality is important for visualizing brain anatomy and pathology and can help inform clinical decisions,” said Wang, who is also a member of the Biomedical Research Imaging Center. “Our generative AI models can perform more accurate and reliable analyses of brain structures, which is critical for early detection, diagnosis, and monitoring of neurological conditions,” said Wang.
Before an MRI can fully process images, it must first remove the bones surrounding the brain (skull) and other non-brain tissue from the images. This process, termed “skull-striping,” allows radiologists to view brain tissue unobstructed. However, MRIs often struggle with producing accurate and consistent results when scanning data is always coming from different types of scanners, individuals, and formats.
Skull-striping, in particular, has a hard time isolating the brain from the skull when the brain goes through dynamic changes, like brain size and inverted tissue contrast between white matter (WM) and gray matter (GM), across the lifespan. As a result, skull-striping might inadvertently remove too much or too little non-brain tissue surrounding the brain, interfering with accurate interpretation of the brain anatomy.
A new paper has shown that their skull-striping model can more accurately remove non-brain tissue and predict lifespan changes in brain volume. Using a large and diverse dataset of 21,334 lifespans acquired from 18 sites with various imaging protocols and scanners, researchers confirmed that their model can faithfully chart the underlying biological processes of brain development and ageing. Limei Wang, a PhD candidate in the Wang lab, was lead author on the paper.
The second AI model, named Brain MRI Enhancement foundation (BME-X), was built to improve overall imaging quality. A previous paper, first authored by Yue Sun in the Wang lab, laid out the specifics of the quality-enhancing model and the ways it can be used to improve patient care and neurological research.
Like their skull-striping model, BME-X was tested on over 13,000 images from diverse patient populations and scanner types. Researchers found that it outperformed other state-of-the-art methods in correcting body motion, reconstructing high-resolution images from low-resolution images, reducing grainy noise, and handling pathological MRIs.
One of its most notable feats is the model’s ability to “harmonize” images from different MRI scanners. There are various MRI scanners in use across clinics and around the country and the world, including those produced by Siemens, GE, and Philips, and each uses different models and imaging parameters.
This variability can make it difficult for clinicians and researchers to have clear and consistent results. BME-X can take in all of the data and level the playing field, creating “harmonized” data to be used for clinical or research needs.
Both AI models have the potential to facilitate clinical trials and studies involving multiple research institutions or MRI scanners. In the field of neuroimaging, the models can also be used to help create new, standardized imaging protocols and procedures. They can also be applied to other imaging modalities, such as CT scans.