GPT-4 Matches Radiologists’ Performance Detecting Errors in Radiology Reports
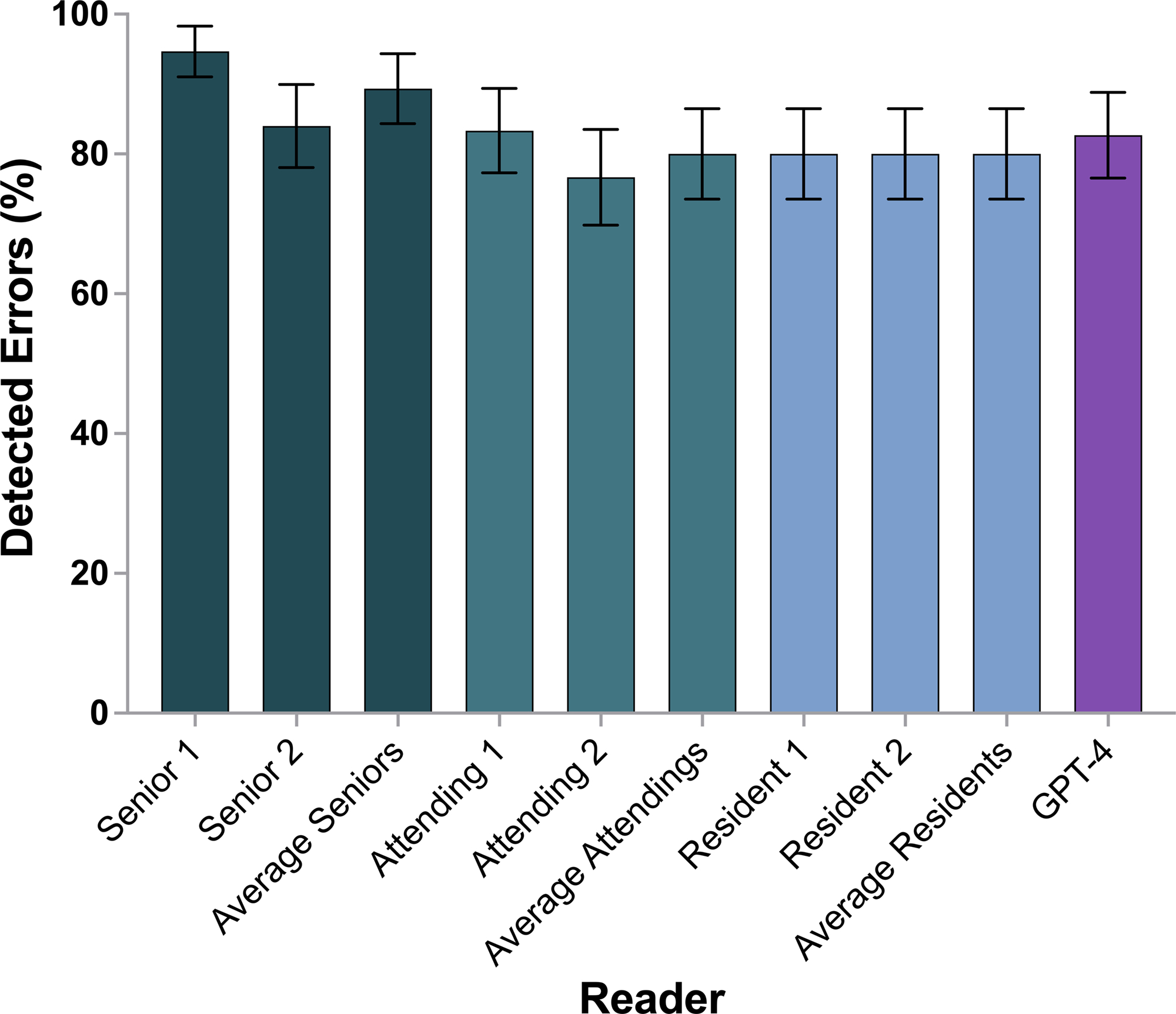
The GPT-4 large language model matched the performance of radiologists in detecting errors in radiology reports, according to research published in Radiology.
Errors in radiology reports may occur due to resident-to-attending discrepancies, speech recognition inaccuracies and high workload. Large language models, such as GPT-4, have the potential to enhance the report generation process.
“Our research offers a novel examination of the potential of OpenAI’s GPT-4,” said study lead author Roman J. Gertz, MD, resident in the Department of Radiology at University Hospital of Cologne, in Cologne, Germany. “Prior studies have demonstrated potential applications of GPT-4 across various stages of the patient journey in radiology: for instance, selecting the correct imaging exam and protocol based on a patient’s medical history, transforming free-text radiology reports into structured reports or automatically generating the impression section of a report.”
However, this is the first study to distinctively compare GPT-4 and human performance in error detection in radiology reports, assessing its capabilities against radiologists of varied experience levels in terms of accuracy, speed and cost-effectiveness, Dr Gertz noted.
Dr Gertz and colleagues set out to assess GPT-4’s effectiveness in identifying common errors in radiology reports, focusing on performance, time and cost-efficiency.
For the study, 200 radiology reports (X-rays and CT/MRI imaging) were gathered between June 2023 and December 2023 at a single institution. The researchers intentionally inserted 150 errors from five error categories (omission, insertion, spelling, side confusion and “other”) into 100 of the reports. Six radiologists (two senior radiologists, two attending physicians and two residents) and GPT-4 were tasked with detecting these errors.
Researchers found that GPT-4 had a detection rate of 82.7% (124 of 150). The error detection rates were 89.3% for senior radiologists (134 out of 150) and 80.0% for attending radiologists and radiology residents (120 out of 150), on average.
In the overall analysis, GPT-4 detected less errors compared with the best performing senior radiologist (82.7% vs 94.7%). However, there was no evidence of a difference in the percentage of average performance in error detection rate between GPT-4 and all the other radiologists.
GPT-4 required less processing time per radiology report than even the fastest human reader, and the use of GPT-4 resulted in lower mean correction cost per report than the most cost-efficient radiologist.
“This efficiency in detecting errors may hint at a future where AI can help optimize the workflow within radiology departments, ensuring that reports are both accurate and promptly available,” Dr Gertz said, “thus enhancing the radiology department’s capacity to deliver timely and reliable diagnostics.”
Dr Gertz notes that the study’s findings are significant for their potential to improve patient care by enhancing the accuracy of radiology reports through GPT-4 assisted proofreading. Demonstrating that GPT-4 can match the error detection performance of radiologists—while significantly reducing the time and cost associated with report correction—this research shows the potential benefits of integrating AI into radiology departments.
“The study addresses critical health care challenges such as the increasing demand for radiology services and the pressure to reduce operational costs,” he said. “Ultimately, our research provides a concrete example of how AI, specifically through applications like GPT-4, can revolutionize health care by boosting efficiency, minimizing errors and ensuring broader access to reliable, affordable diagnostic services—fundamental steps toward improving patient care outcomes.”