Fighting Obsolescence: Professional Assessment in the Era of ChatGPT
By Berland LL, Hardy SM
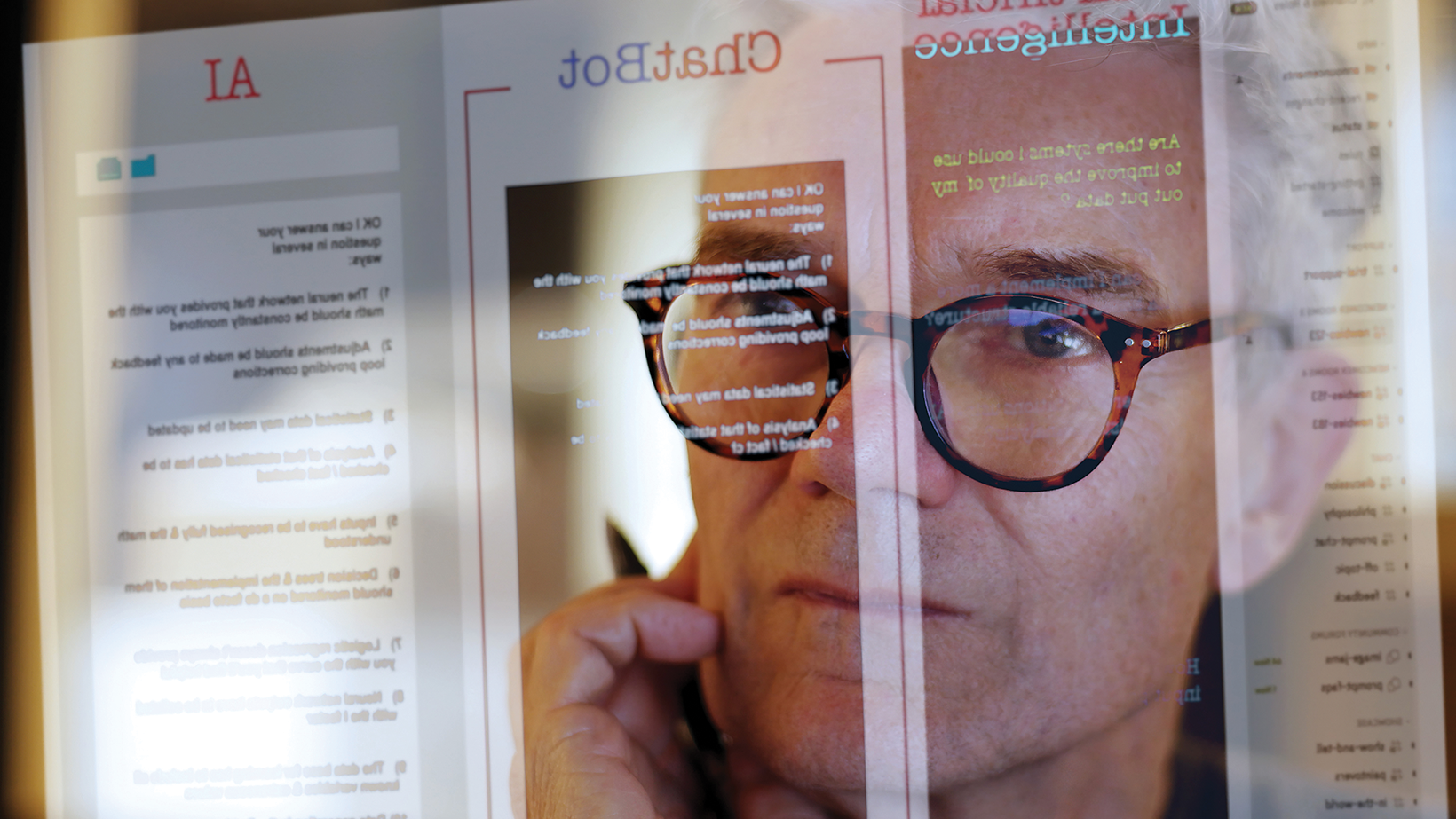
The recent release of ChatGPT, a natural language processing technology developed by San Francisco, CA-based OpenAI.com, has excited the public with its promise of disruptive innovation.
With its startling ability to answer complex questions coherently (often erroneously), ChatGPT— and competing large language model (LLM) deep-learning (DL) algorithms— has also captured the attention of healthcare leaders, many of whom envision exciting opportunities for its application to physician education and practice.
ChatGPT, however, is not without its risks, particularly with respect to radiology education and assessment. Indeed, the technology’s capacity to “pass” professional assessment examinations threatens to make conventional tests obsolete. To head off this threat, radiology leaders must begin preparing now to replace current examinations with alternative, “authentic” assessment methods that simulate clinical practice and more fully address the broad range of skills required for professional competence.
ChatGPT: Surprising in More Ways than One
Despite the many obvious, rapid advances in artificial intelligence (AI) in recent years, and preexisting systems based on earlier, similar algorithms, the arrival of ChatGPT came as quite a surprise to many in the general public and specialized fields alike. The algorithm also has a startling ability to generate sophisticated, human-like responses to a head-spinning scope of questions and challenges; ChatGPT can recommend a good local restaurant, plan a schedule, describe complex physiologic phenomena, and even compose radiology reports, to name just a few of its many capabilities. Upon its release in November of 2022, ChatGPT attracted over a million users in five days, and 100 million in two months, smashing records for software adoption.
Microsoft, Google, and Meta all have or will be deploying and refining similar algorithms in upcoming months and years. Other quickly-evolving AI systems already generate astonishingly realistic, “deep fake” images, videos, audio, and music. Just as it is essentially impossible for a human to visualize the vast distance between planets, stars, and galaxies, humans are also incapable of fully appreciating the scope and volume of data used to construct these LLMs, or the speed at which they can process this information.
However, while the broad capabilities of these systems may even appear mystical, our inability to fully understand how they work should not translate to incorrectly concluding, as some have, that they are sentient.
ChatGPT is an artificial (or augmented) intelligence algorithm (also termed a “chatbot”) that uses DL, one class of machine learning that involves training artificial neural networks to learn representations of data. The acronym GPT stands for “Generative Pretrained Transformer,” which is a chat-capable interface based on an LLM and designed to process and generate natural language.1 Its initial training is “unsupervised;” ie, analyzed without human input or annotation.
GPTs are built upon “Transformer,” a DL neural network architecture1,2 that converts a continuous sequence of text into discrete units, called tokens, and then analyzes patterns and context to, for example recognize multiple uses for single words. A “self-attention” mechanism weights the importance of different words in a sentence, helping to predict the next most likely word in its response based on the prompt provided. The pretraining database derives from a wide variety of sources such as web pages, books, articles, forums, and other publicly available documents, but is current only up to 2021 as of this writing.
Partly because this pretraining includes large volumes of human-generated text from fiction, contradictory and contentious online discussions, and misinformation, the output of a GPT can include dramatic, personal, shocking, racist, and factually erroneous statements, often stated with infuriating, and misplaced, confidence.2 ChatGPT can also generate “AI hallucinations” or “stochastic parroting” – incorrect, fabricated, or nonsensical output – that can be caused by either limitations and biases in the training data or the failure to fully appreciate context.
To improve performance, accuracy, and appropriateness of responses, human feedback is applied to the algorithm. The features and abilities of these systems are developing dynamically and there is little question that they will become considerably more impressive and reliable in upcoming versions and in competing products.
Humans are biased to imbue computer-simulated interactions with human characteristics, even though they do not arise from human senses, experiences, observations, and reasoning.2 The output of GPT tends to lead to anthropomorphizing these systems when they are actually based only on statistical analysis of language. For example, their answers use the first person “I” to indicate their actions and “understanding” to imply that they use reasoning; and their responses are grammatically and syntactically coherent, giving one the very strong impression they are conversing with a live individual online.
This anthropomorphizing presents the risk of “authority bias,” in which people tend to accept outcomes without question. Another risk is that of “confirmation bias,” where a statement is accepted because it plausibly fits a preexisting expectation. A further risk is simply that of seeing the GPT as a shortcut because of how well and quickly it retrieves information for which a human would have to perform time-consuming research. So, on the one hand, we may resist using this algorithm, and on the other, we may be tempted to use it too uncritically.
Indeed, integrating this software into medical use will require discriminating, thoughtful analysis. While the technology is likely to provide meaningful support to next-generation learners, its implications for radiology training and assessment are significant and should be fully appreciated before the field as a whole goes all-in on ChatGPT and its technological siblings.
For example, multiple-choice question (MCQ)-based board examinations are long-accepted standards, although their validity and utility have been challenged.3-5 Recent experiments have found that ChatGPT can approach a passing score on an examination structured to resemble the United States Medical Licensing Examination (USMLE) but modified to exclude images and graphs.6 ChatGPT has also performed surprisingly well on an examination that simulated a portion of the United Kingdom’s Fellow of Royal College of Radiology examination.7 While these LLMs have not yet overtly passed such tests, these early exercises raise the question as to whether our standard examinations test a sufficiently broad array of skills required to be a competent physician. Augmented Intelligence systems, including such LLMs, are rapidly proliferating and improving, beginning to integrate images and other media, using much larger data sets, and linking to real-time online resources.6 Consequently, their ability to convincingly pass professional examinations seems likely in the near future.
Should we allow computer applications to act as physicians? Rather than ponder this absurdity, we may analyze the weaknesses of our current forms of education and certification in the context of AI. Competent physicians can no longer compete with AI in fact-extraction and probabilistic judgments. However, they can perform physical examinations and procedures; detect abnormalities (including rare entities for which no large data sets exist); assemble, interpret, and prioritize information; collaborate; generate conclusions and recommendations; report findings; communicate the importance of information to patients and physicians; show empathy; and demonstrate professionalism, ethics, and leadership. We question the utility of examinations that fail to test any of these skills.
So, if AI algorithms may soon be able to pass some existing professional examinations but remain unable to demonstrate the core skills necessary for clinical competence, are the American Board of Radiology (ABR) Core and Certifying Examinations and Continuing Certification (CC) tests adequate to serve, as the ABR demands, “patients, the public, and the medical profession by certifying that its diplomates have acquired, demonstrated, and maintained a requisite standard of knowledge, skill, understanding, and performance”?
The evidence to answer this question is sparse; however, the validity and utility of MCQ Board examinations were already being questioned prior to the advent of this disruptive technology.3-5 Furthermore, standardized testing itself in higher education has been widely criticized,8-10 including by an organization (Fairtest.org) devoted to educating the public on problems with standardized testing.
In addition, a stakeholder input survey by the ABR itself has found that “multiple choice questions can adequately (though not optimally) assess knowledge, but the overall process is a poor measure of clinical competence as it pertains to interpretation skills, communication skills, and professionalism.”11 Residency programs monitor a broad set of milestones, and they must affirm that residents are qualified to take their certifying examinations, but they perform no comparable end-of-training assessment. We believe that using an MCQ test as an exclusive “final exam” is obsolete in this era of such increasingly powerful AI tools. In response to its stakeholder survey and other input, the ABR on April 13, 2023, announced that it will convert the Diagnostic Radiology Certifying examination to an online “oral boards” format in 2028.12 However, for the moment, the other examinations are left unchanged.
Even before the emergence of this new application of AI, an American College of Radiology membership survey found that only 1.7% of respondents considered the ABR regimen of CC requirements acceptable.5 Given these results, many expect better scientific evidence of the examinations’ effectiveness and that they should be more adaptable to the broadly varying practices of radiologists.5 The ABR argues that “the oral exam aims to assess higher-level skills that are needed to be an effective diagnostic radiologist and are valued by referring physicians and patients.” However, the ABR has not yet clearly explained how the ABR will adapt to the threats posed by ChatGPT to written/MCQ exams.12
Radiology’s value within the healthcare ecosystem is almost entirely dependent upon payers’ willingness to pay (WTP).13 Radiologists’ value is not a variable over which referring physicians or patients have much influence, given that they are ancillary stakeholders in any radiology value paradigm and have little control over payers’ WTP in today’s siloed care networks.13 Currently, competence as certified by the USMLE and the ABR is recognized by state medical licensure boards, healthcare institutions, courts, and insurance payers as a threshold for WTP. But now that ChatGPT has exposed the vulnerabilities of MCQs for professional assessment, physicians may risk losing their value unless the examinations pivot to a new paradigm.
What alternatives to support WTP could be considered? Modern educational theory points to “authentic testing” as a better means to establishing competence.
Authentic testing can be based on simulations of actual clinical practice. The previous oral board examination format, imperfect as it was, was the closest radiology had to modern simulation techniques, and we are hopeful that the new oral examination will be restored using principles of authentic assessment. Already, robust simulation-based, authentic-testing assessments have been developed and extensively validated in 68 unique Accreditation Council for Graduate Medical Education programs with over 1700 residents during the past ten years.15, 16 These are proven efficient and effective at assessing peer competency by subspecialty with eight-hour shift simulation, including normal cases.
As educators know, what students learn is strongly influenced by the knowledge and skills that are tested. Future professional assessment must build upon radiologists’ unique human strengths, including those of collaboration, empathy, curiosity, learning without the need for large data sets, and the ability to apply innovative analysis.
Artificial intelligence will increasingly be able to compensate for radiologists’ weaknesses by leveraging growing data sets in biology and pathophysiology to provide immediate access to the information they require. However, AI can never fully understand meaning, apply knowledge, or empathize with unique humans in unique situations. It is strategically imperative for physicians to recognize that human uniqueness and tailor their treatment of each patient accordingly. Ultimately, competent physicians of the future will need to artfully synergize AI with their own experience to serve their patients.
In the meantime, radiology organizations must make the transition to authentic methods of professional assessment and adopt the new AI technologies in order to avoid the obsolescence that threatens to arrive more quickly than we all may expect.
References
- Shen Y, Heacock L, Elias J, et al. ChatGPT and other large language models are double-edged swords. Radiology. 2023; 307(2):e230163. https://doi. org/10.1148/radiol.230163.
- Kissinger H, Schmidt E, Huttenlocher D. ChatGPT heralds an intellectual revolution. Wall Street Journal. Feb. 24, 2023. https://www.wsj.com/articles/ chatgpt-heralds-an-intellectual-revolution-enlightenment-artificial-intelligence-homo-technicus-technology-cognition-morality-philosophy-774331c6. Accessed 4-19-23.
- Berland LL, Berland NW, Berland MW. ABR psychometric testing: analysis of validity and effects. J Am Coll Radiol. 2018;15:905-910. https://doi.org/10.1016/j. jacr.2018.02.023.
- Berland LL, Heitkamp DE, Beavers KM, et al. Report of the ACR Task Force on Certification in Radiology: History, Challenges and Opportunities. https:// www.acr.org/Lifelong-Learning-and-CME/ACR-Report-on-Certification-in-Radiology. Accessed 4-19-23.
- Berland LL, Tarrant MJ, Heitkamp DE, Beavers KM, Lewis MC. Maintenance of certification in radiology: eliciting radiologist preferences using a discrete choice experiment. J Am Coll Radiol. 2022;19:1052-1068. https://doi.org/10.1016/j.jacr.2022.06.012
- Kung TH, Cheatham M, ChatGPT et al. Performance of ChatGPT on USMLE: potential for AI-assisted medical education using large language models. medRxiv December 21, 2022. doi: https://www.medrxiv.org/content/10.1101/2022.12.19.22283643v2.full.pdf. Accessed 4-19-23.
- Elmahdy M, Sebro R. Beyond the AJR: comparison of artificial intelligence candidate and rdiologists on mock examinations for the Fellow of Royal College of Radiology Part B. AJR 2023 published online. doi:10.2214/AJR.23.29155
- Ravitch D. Lessons learned. In: Ravitch D, ed. The death and life of the great American school system; how testing and choice are undermining education. New York, NY: Perseus Books Group; 2010:223-42.
- Wiggins G. A true test: toward more authentic and equitable assessment. Phi Delta Kappan. 2011;92:81-93.
- Dalal J, Gunderman RB. Standardized tests: a review. J Am Coll Radiol 2011;8:271-4. doi:https://doi.org/10.1016/j.jacr.2010.08.006.
- Barr RM. ABR Update. Presentation at Texas Radiological Society, Austin, TX, 2-17-22.
- Transition to New DR Oral Exam. https://www.theabr.org/news/new-diagnostic-radiology-oral-exam. Accessed 4-19-23.
- Hardy SM. Value chain: where radiologists should put their focus in threats against income. Appl Radiol. 2021;50(4):32-34. https://appliedradiology.com/ articles/value-chain-where-radiologists-should-put-their-focus-in-threats-against-income.
- United States, Federal Aviation Administration, Department of Transportation. Flight review. 14 Fed Reg. 61.56.
- Sistrom CL, Slater RM, Rajderkar DA, Grajo JR, Rees JH, Mancuso AA. Full resolution simulation for evaluation of critical care imaging interpretation; Part 1: fixed effects identify influences of exam, specialty, fatigue and training on resident performance. Acad Radiol https://doi.org/10.1016/j.acra.2019.11.023.
- Sistrom, CL, Slater RM, Rajderkar DA, Grajo JR, Rees JH, Mancuso AA. Full resolution simulation for evaluation of critical care imaging interpretation-art 2: random effects reveal the interplay between case difficulty, resident competence, and the training environment. Acad Radiol. 2019; https://doi.org/10.1016/j. acra.2019.11.025.