AI Technique for MRI May Guide Real-Time Glioblastoma Treatment
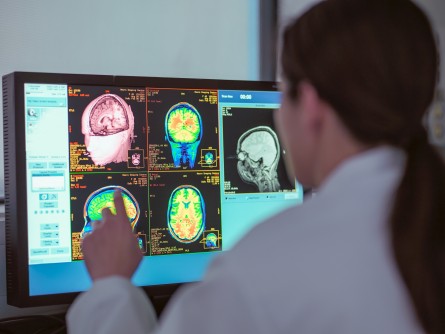
Researchers from Sylvester Comprehensive Cancer Center, part of the University of Miami Miller School of Medicine, are working to shed light on whether a patient’s glioblastoma has responded to ongoing treatment or progressed, using a system known as MRI-guided radiation therapy that pairs daily MRIs with radiation treatment. These machines use magnetic resonance imaging to light up the brain tumor and guide the directed radiation beams, but because MRI captures so many details, the daily imaging also holds the potential to provide near real-time insight into how a patient’s tumor is progressing or responding.
“We’re getting this time resolution of MRIs in glioblastoma patients that nobody’s ever seen before and we’re trying to figure out, what is that useful for?” asked Eric A. Mellon, MD, PhD, co-leader of Sylvester’s Neurologic Cancer Site Disease Group and a radiation oncologist, who leads research on brain cancer with MRI-guided radiation therapy. “If the glioblastoma is changing one way or the other, what does that mean for the eventual outcome of the patient?”
To answer these questions, Dr Mellon and his colleagues have to contend with a huge amount of data generated by the emerging technology. For each of the 36 glioblastoma patients in their study, the method generated 31 timepoints, each containing four to six different images. To help sift through these extensive datasets, the Sylvester researchers, led by Dr Mellon and Radka Stoyanova, Ph.D., director of imaging and biomarkers research at Sylvester, turned to artificial intelligence.
The scientists developed a machine-learning approach to automatically trace the glioblastoma tumors and resection cavities, the space left in the brain when tumors are surgically removed, in these large MRI datasets. They published an article describing their work in the journal Cancers.
These automatic traces, outlining the edges of a tumor in the brain, can tell physicians whether a tumor is growing or shrinking during the course of treatment. The algorithms, which the team adapted from earlier work automatically tracing cervical cancer, capture the precise volume of the tumor and how that volume changes over time.
They’re also a huge time-saver. Even for a trained expert, manually outlining the tumor in MRI images can take upward of 20 hours per patient, due to the sheer number of images the technique generates. The machine learning method can churn through that data in about 90 minutes, said Kaylie Cullison, an MD/PhD student in the Miller School’s Medical Scientist Training Program and member of Dr Mellon’s lab who was co-first author on the new study along with Adrian Breto, a doctoral student and programmer in Stoyanova’s laboratory.
“This project was really tailor-made for deep learning,” Dr Stoyanova said. “You really can’t do this work otherwise, unless you have a team of clinicians manually tracing images around the clock. The MRI-guided radiation therapy is a great place to apply these new machine learning techniques.”
The newly published machine learning approach deals primarily with tracing a tumor’s volume and edges, as well as those of the resection cavity, but the research team wants to expand these applications to capture other information from the image dataset as well. One particular area of focus for the glioblastoma team is a phenomenon known as pseudo-progression, where the tumor appears to be growing but is actually swelling in response to treatment and will eventually die off. Determining true progression from pseudo-progression in MRI images is a tricky but important area of research.
“There’s so much information in these images,” Cullison said. “The sky’s the limit.”
Dr Mellon and his team are currently working on designing a study where physicians will assess glioblastoma tumor progression weekly in patients undergoing MRI-guided radiation therapy and adapt their treatment if the tumors are not responding to the standard therapy or if radiation positioning needs to be changed due to changes in the size of the tumor. They’re planning to use the new machine learning method to enable this rapid adaptation of treatment.
“You can monitor so many different qualities of the tumor with MRI. That’s an untapped frontier,” Breto said. “We haven’t gone yet to the center of the earth as far as what MRI can tell us about the patient’s disease and quality of life. That’s what we’re trying to do, squeeze as much information as we can out of these images for the benefit of the patient.”