AI’s Diversity Problem in Radiology: Addressing Algorithm Bias
By Reeves K
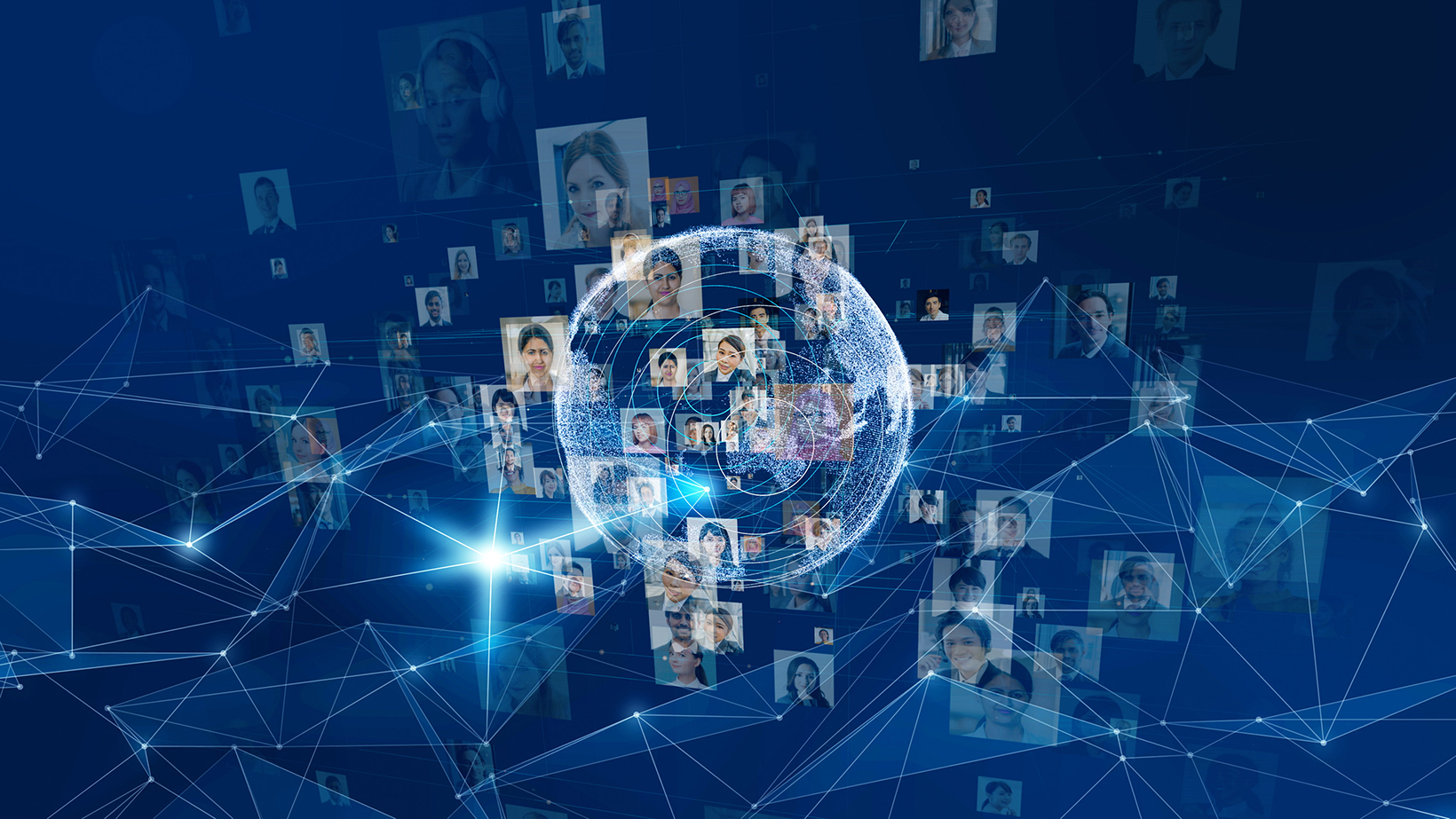
As the volume and breadth of healthcare data continue to expand, so too are the opportunities to apply artificial intelligence-based solutions (AI) to a growing number of medical tasks, including many in radiology.
However, care must be taken, many experts say, to ensure that the promise of AI reaches all patients, regardless of race, gender, and other demographics, by making it a priority to train the underlying algorithms of AI solutions on as many diverse patient populations as possible.
The AI Toolkit is Growing
More than 520 AI-based medical algorithms cleared by the US Food and Drug Administration (FDA) are helping to make diagnoses, treatment recommendations, and health outcomes predictions.1 They are also streamlining administrative functions related to billing, patient records, and pre-authorizations.
In radiology, nearly 400 dedicated AI-based algorithms are being applied to spot potentially cancerous lesions, to advance image processing tasks, to generate 3-D models, and to assist in generating reports. 1
Yet, while the potential of AI to continue improving upon medical imaging is promising, concerns are being raised about bias— specifically with respect to bias in the datasets used to train AI solutions in healthcare.
“We need datasets that represent the beautiful diversity of our patients, whether that’s gender, ethnicity, age, or any other type of diversity,” says K. Elizabeth Hawk, MS, MD, PhD, assistant professor at the Stanford School of Medicine, interim chief of health sciences, and associate clinical professor of nuclear medicine at the University of California San Diego. “Otherwise, [algorithms] may underperform for the under-represented patient populations.”
Dr Hawk and Sonia Gupta, MD, chief medical officer of Enterprise Imaging at Optum and a radiologist specializing in oncology, shared their thoughts on the need for more diverse AI-training datasets in an interview with Applied Radiology editor-in-chief Erin Simon Schwartz, MD, at RSNA 2023 in Chicago. Their conversation followed a panel discussion at the meeting.2
To avoid bias, patient harm, and discrimination in the provision of care, the data used to train AI algorithms must include the full range of patient gender, ethnicity, race, age, and geography, as well as any with genetic predispositions to certain diseases and/or issues with access to healthcare services, says Dr Gupta.
For example, she argues, an algorithm designed for cancer detection should incorporate data from patients with reliable access to regular screening, as well as from those who do not have those advantages. These populations commonly include ethnic and racial minorities, women, and children, among others, who may be excluded from the datasets used to train computer programs.
Dr Gupta cites age diversity as a particular concern as it relates to identifying and meeting the healthcare needs of children. “[We need] more algorithms that are developed exclusively for pediatrics because we [often] go backwards in that we start with adults and then hope that we can retrofit it to children, but it’s not the same,” Dr Gupta says. “Children are not little adults … . [They have] completely different physiology and disease processes.”
“It’s important to bring pediatric radiologists to that design table,” adds Dr Hawk. “A lot of these AI development teams have been focused on adult algorithms … and when they start looking to develop more pediatric algorithms, it’s important to bring radiologist voices to the design process.”
“When we don’t have diversity of data … it actually deepens healthcare disparities across the globe, not only for our patients, but also for our provider teams,” she says.
With respect to geographic diversity, Dr Hawk argues that US healthcare would greatly benefit by doing more to integrate rural populations in the training of AI algorithms. The same is true for improving gender diversity; she notes that algorithm re-approvals through the FDA provide an opportunity to “tune-up” existing algorithms.
“That’s a good time to look at the blind spots like gender diversity and make sure that the new datasets include a wider gender diversity, depending on the algorithm [and its needs],” Dr Hawk says.
AI Bias in Healthcare Coming Under Scrutiny
The impact of bias on healthcare AI has captured the attention of legislators. In November, The Senate Health, Education, Labor, and Pensions Committee held a hearing on policy considerations for AI in healthcare, and The House Energy and Commerce Subcommittee held one on considerations for Congress as AI evolves. Participants shared concerns about inequitable use of AI that could exacerbate health disparities.3
US Sen. Ben Ray Luján of New Mexico noted that AI data gathered mostly from male patients performed poorly when physicians applied it to female patients. Sen. Luján also pointed to an algorithm designed to diagnose skin cancer trained on lighter-skinned patients that would fail on darker-skinned people.3
A study addressing patient health management and published in the journal Science also showed that algorithms used in healthcare are racially biased.4 This study found “large racial biases” in the prediction of healthcare costs over illness resulting from unequal access to care. In addition, market forces and pre-existing societal prejudices of the data itself also play a role in the under-representation of certain populations, according to a recent article in the Harvard Business Review.5
Overcoming bias in the development of healthcare algorithms is challenging. Many algorithms are proprietary, and humans often cannot know specifically what pieces of information are used by a given AI-based program to make recommendations, how those data are weighted by the program, or even what data are included or excluded.6
Developers and users alike largely cannot reason through AI’s “decisions.” As disparities arise, there is a risk of patterns being repeated, resulting in the further amplification of existing inequities.
For example, one study that examined algorithmic underdiagnosis in the classification of pathologies across three large chest X-ray datasets and a multi-source dataset found that classifiers produced using state-of-the-art computer vision techniques consistently and selectively underdiagnosed certain underserved patient populations. The study also found that the underdiagnosis rate was higher for intersectional underserved subpopulations such as, for example, Hispanic female patients.7
The researchers concluded that the deployment of AI systems with such biases for medical imaging-based diagnosis risks worsening existing care biases and leading to unequal access to medical treatment.7
Improving Data Diversity
While developers are limited by the availability of diverse datasets and technological aspects of algorithm training, there are ways to reduce AI bias in medicine. Focusing on diversity within algorithm development teams, including members’ age, race, gender, and geography, can help to ensure data representation across populations, Dr Hawk says.
“Really look at the [development] team … and ask, ‘will this represent the diversity of my practice or where I want my practice to go?’” Dr Hawk says. “If [the company] has a homogeneous team with homogeneous minds problem-solving … around how to create an algorithm, then they’re going to design something that looks and feels like a solution for their problems.” She adds that this can adversely impact the providers who use the technology and their patients whose disparities are deepened by its use.
Increased diversity of a development team also can result in a comprehensive evaluation of technology performance for every user, Dr Hawk says. She cites the example of an employee for whom English is a second language benefiting from a natural language processing algorithm.
“If you have diversity in your team, they will [ensure] that diversity was created in the design process of the algorithm,” she says. Improving diversity, equity, and inclusion in radiology, as a whole, is long overdue, says Dr Gupta, who notes the under-representation of minorities and women in the specialty. Statistics show that only 23% of radiologists are women; only 1.7% are Black; and 3.7% are Hispanic or Latino, compared to 6.2% and 5.3% of medical school graduates overall, and 13% and 18% of the population, respectively.8
“[Our] specialty is a leader in healthcare in developing AI, and if our trainees, residents, and fellows don’t reflect the diversity of the general population, then we’re not going to reflect more diversity as we develop AI and get these algorithms into the market,” Dr Gupta says. “Going to the source—the radiologists who are becoming leaders in the AI space within healthcare—is really important.”
Involving a more diverse body of stakeholders in training, reviewing, and supervising development of the algorithms, and validating the data, will help address bias issues within healthcare AI.
Leaders must “guide the needle into a better direction that lessens healthcare inequity, improves diversity across our field, [and] really places an element of empathy, kindness and patient-centered care into the work that we’re doing,” Dr Hawk says.
References
- Fornell D. FDA has now cleared more than 500 healthcare AI algorithms. Health Exec. Feb 6, 2023. Accessed via https://healthexec.com/topics/artificial- intelligence/fda-has-now-cleared-more-500-healthcare-ai-algorithms.
- AI: The Importance of Diversity in Data. 2020. Applied Radiology, accessed via https://appliedradiology.com/articles/ai-the-importance-of-diversity-in-data.
- Health Subcommittee Hearing: “Understanding How AI is Changing Health Care.” Nov. 29, 2023. House Committee on Energy & Commerce, accessed via https://energycommerce.house.gov/events/health-subcommittee-hearing-understanding-how-ai-is-changing-health-care.
- Obermeyer Z, Powers B, Vogeli C, Mullainathan S. Dissecting racial bias in an algorithm used to manage the health of populations. Science. 2019; 366(6464): 447-453. Doi: 10.1126/science.aax23.
- Friis S, Riley J. Eliminating algorithmic bias is just the beginning of equitable AI. Harvard Business Review. Sept 29, 2003. Accessed via https://hbr. org/2023/09/eliminating-algorithmic-bias-is-just-the-beginning-of-equitable-ai#:~:text=Algorithmic%20bias%20often%20occurs%20because,baked%20into%20 the%20data%20itself.
- Sharfstein J. How health care algorithms and AI can help and harm. Johns Hopkins Bloomberg School of Public Health. May 2, 2023. Accessed via https:// publichealth.jhu.edu/2023/how-health-care-algorithms-and-ai-can-help-and-harm.
- Seyyed-Kalantari L, Zhang H, McDermott M, et al. Underdiagnosis bias of artificial intelligence algorithms applied to chest radiographs in under-served patient populations. Nat Med. 2021; 27, 2176-2182. https://doi.org/10.1038/s41591-021-01595-0
- Omofoye T, Bradshaw M. The emerging diverse radiology workplace: case studies on the importance of inclusion in radiology training programs, Acad Rad, 2023; 30:(5) 983-990. ISSN 1076-6332, doi: https://doi.org/10.1016/j.acra.2022.05.012