AI Model for Identifying Recommended Imaging in Radiology Reports Outperforms Traditional Machine-Learning Model
Images
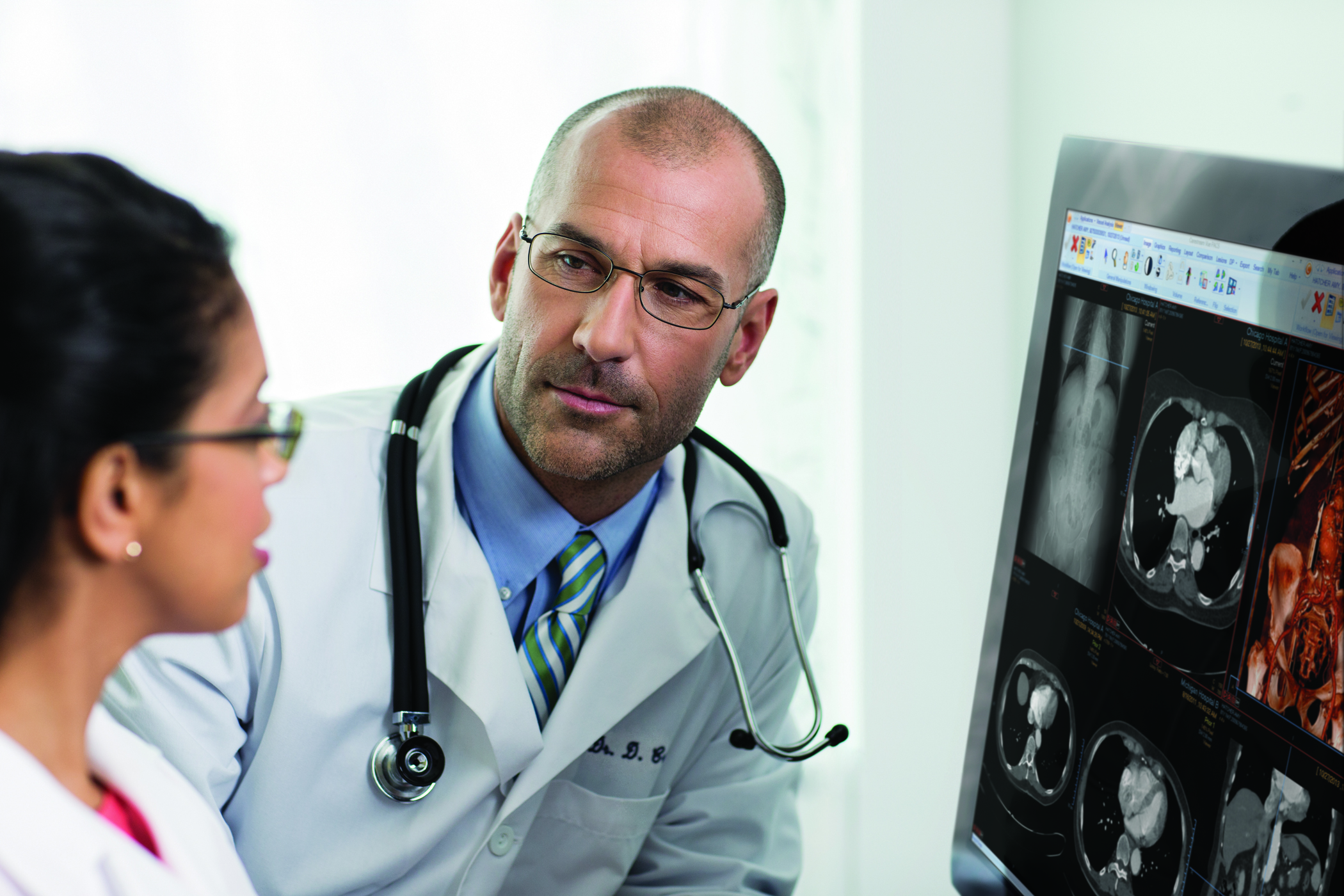
A study published in the American Journal of Roentgenology found that a Bidirectional Encoder Representations from Transformers (BERT) deep-learning model outperformed a traditional machine-learning (TML) model in accurately identifying radiology reports with recommendations for additional imaging (RAI).
The retrospective study, performed at a multi-site health center, included 6300 reports randomly selected from one center and another 1260 randomly selected for external validation from another center. Varying subspeciality radiologists manually reviewed the reports for instances of RAI.
The BERT-based AI model achieved an accuracy score of 99% in identifying reports with RAI compared to 93% accuracy for the TML model in prior studies, suggesting it could be applied for real-time EHR monitoring for more timely clinically recommended follow-up. High external validation of 99% further suggests the model can be applied to other health systems without requiring institution-specific training.
According to the authors, the BERT-based model required minimal pre-processing, or the extraction of the radiology report’s impression section, and performed the evaluation rapidly. They suggest that the model could be applied along with automated workflows to identify whether the recommended imaging was performed and to generate reminders when the RAI was not performed.
The code for the model is publicly available at: https://github.com/NooshinAbbasi/Recommendation-for-Additional-Imaging