Of AI, Radiology, and Africa
Images
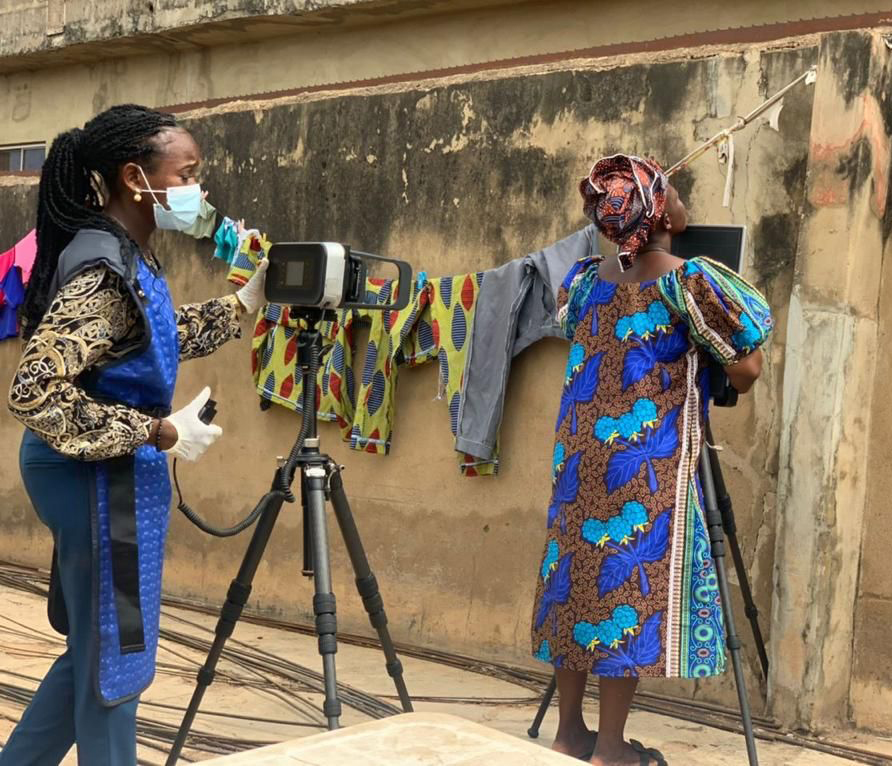
During “AI after Dark,” a discussion at RSNA 2018 about how artificial intelligence (AI) will change radiology, the mood was typically jubilant. We radiologists felt good about ourselves, and it wasn’t just the whisky. We were discussing how AI could solve Africa’s shortage of radiologists.
But suddenly the mood changed.
“You speak about AI,” said Monica Atalabi MD, a pre-eminent Nigerian radiologist and head of radiology at University college hospital, Ibadan. “In Nigeria, we don’t even have PACS!”
The crowd looked embarrassed. I, the moderator, felt like a modern-day Marie Antoinette and bit my nails.
After Geoffrey Hinton, the pioneer of machine learning (ML), famously announced the imminent extinction of radiologists at the hands of AI, entrepreneurs put their money where their delusions were. A thousand companies were launched on a mission to replace radiologists. One venture capitalist, Vinod Khosla, even quantified the degree of replacement. Eighty percent of doctors, Khosla predicted, would be replaced by AI algorithms.
British radiologist Hugh Harvey has a graph comparing the number of AI start ups that no longer exist to the number of radiologists replaced by AI. The former is represented by a tall histogram that grows exponentially taller every year; the latter, by the number 0. Jokes aside, the problem is that AI was framed under the capitalistic, Silicon Valley, “Hey, ho, let’s disrupt healthcare” mindset, a mindset that drove away its largest potential customers – radiologists.
When it became clear that Hinton was no Nostradamus, the narrative on AI’s impact shifted. It was no longer the money that would be saved by replacing radiologists; it was the money that would be made by “optimizing” radiologists. AI would help us work faster, crunch more RVUs, render fewer errors, and comply more closely with guidelines.
I don’t know about you, my fellow radiologists, but the thought of AI helping me read 20 more chest radiographs per hour is as exciting as the annual billing-compliance meetings that I attend. AI has gone from a brute, amoral disrupter to a domesticated pet technology; to yet another incremental innovation intended to help adminstrators dance at the margins of an overoptimized system.
At the 2022 Association of Radiologists in Nigeria meeting in Lagos, teleradiology and AI were the dominant themes. The juxtaposition wasn’t coincidental. Radiologists were initially suspicious of both technologies, seeing them as means of rendering their professional skills less relevant. Teleradiology, at least, has since proven to be the opposite. But the plot twist that few anticipated was that both technologies now actually promote healthcare equity by bringing radiological expertise to underserved communities.
So, how can AI help Africa? To answer this question, we need to reframe AI as an enabler of radiology instead of a disruptor of radiology. Let’s start with the imaging equipment. Fundamentally, AI permits reconstruction of images with lower juice. For CT, this translates to the same image quality at a lower radiation dose, or higher-quality images at the same dose.
This sounds like the incremental innovation I derided. But it depends on how the incremental gains are used. Reducing dose from one mSv to 0.8 mSv is a “meh.” But reducing CT power usage by 20 percent in countries like Nigeria, where outages occur daily, is huge.
MRI is more complex than CT, but the “juice” principle is similar: being able to generate images with equal or higher quality with lower Tesla magnets, the sort that historically drove radiologists to despair because of noisier images. MRI’s muscle lies in the strength of the magnet; our benchmark is the 1.5 T magnet. “Tesla” is a unit of magnetic strength named after polymath, Nicola Tesla. A typical MRI is 30, 000 times stronger than the earth’s magnetic field. AI enables image reconstruction with a lower upfront signal, permitting lower-field MRI to work, not perfectly, but diagnostically. MRI machines that don’t capitulate to power outage are an obvious win in Africa. In technical terms, severing the interdependence between power supply and health of MRI means doing without superconducting magnets, which means no need for helium and less fear of the dreaded quenching. Again, incremental improvements in the reconstruction space courtesy of AI lead to dramatic gains in hardware.
Ultimately, it’s up to vendors to translate these gains to cheaper hardware. The means are available, but the will? That depends on the the business case, which depends on price elasticity of demand for equipment, which is arguably greater in Africa than in the US, where elasticity is blunted by third-party payers. Small reductions in price can lead to large increases in sales, which can drive adoption. Africa doesn’t need charity. Africa needs strategic pricing. Vendors, such as Fujifilm, Canon, and Hyperfine, are using this rationale to deliver cheaper equipment to Africa.
Of the three requirements for access—proximity, affordability, and expertise—I would argue that proximity is the most important. It does sick patients no good if they must travel hundreds of miles to for medical imaging. The cost of imaging isn’t just the cost of getting the scan; it’s also the cost of getting to the scan, which often is more than just pecuniary. Increasing access means bringing imaging to the patients.
This concept is hardly revolutionary. During the First World War, Marie Curie’s mobile contraption, known as “Little Curie,” brought X-rays directly to soldiers on the Western Front. A century later, reading rooms have become our redoubt. We radiologists seldom travel to patients. We expect them to come to us, which is fine if you live in the Acela corridor but not so much if you live along the Niger River.
Renowned McGill University tuberculosis researcher Madhukar Pai believes chest X-rays, like basic lab tests, should be part of the essential diagnostic toolkit. X-ray machines are no longer just big, bulky contraptions. They are portable—really portable. Some can even fit in backpacks and be carried to remote locales without an entourage of technical experts. Innocent Ugwoke, manager for Sales for Fujifilm Africa, says portable X-rays are easier to take than selfies.
But this still raises a question: Who will read the scans? Here, Professor Atalabi’s point is salient. Without PACS and cloud technology there’s no central repository for images to be stored for interpretation by radiologists, non-radiologists, or even AI.
But do we even need PACS for AI to work? It turns out we don’t. Algorithms from vendors such as Qure.AI and Lunit are embedded in many portable X-ray machines, so that when images are taken, AI can instantly generate a report. This is an example of conjoint technologies, where the whole is greater than the sum of its parts. They increase each other’s relevance. The portable X-ray allows AI to travel to remote locations, such as Maidugiri in Northern Nigeria; the embedded AI in return obviates the need for PACS and radiologists. Even Nigerian physicians are afraid of traveling to places like Maidugiri, the hub of Boko Haram and where kidnapping still runs rampant. Thankfully, algorithms can’t be kidnapped.
Portable X-ray machines are vectors that can and are transmitting near-radiologist expertise to the masses. “Little Curie” has been revived, and far from replacing us radiologists, AI serves as our representative. In a sense, we all work for Africa. AI has scaled our influence. The future belongs to AI-embedded technology. Dara Kelleher, Director of Public Health Outreach at Qure.AI, predicts AI algorithms will become as ubiquitous in radiologic hardware as Intel microchips are in computers.
Perhaps the most interesting part of the story of algorithms in Africa is their financier. The World Health Organization (WHO) wants to end tuberculosis by 2035. This can’t be achieved without getting X-ray imaging to the masses. Here again, AI can come to the rescue. Lunit, CAD4TB, Deeptek, Qure.AI and other vendors have developed algorithms to detect TB in chest x-rays. Those patients whose scans are flagged by AI as positive are then referred for a confirmatory Xpert gene test. WHO’s wish has galvanized the public health community; money to fight TB is flowing from philantrophic organizations such as the Gates Foundation, USAID, and Global Fund. Public health agencies in Africa have purchased several AI-enabled X-ray machines and, in some instances, the vans to transport them.
The profound irony of all this is that AI is developing not from private money thrown by Silicon Valley venture capitalists to replace radiologists in rich countries, but from public health funding being used to fight TB in the poorest countries. Funding is arising from complex public-private partnerships tasked with eliminating various infectious diseases.
This explains why AI is an equity-promoting technology. It is fundamentally a tool of public health in low and middle income countries. While American radiologists fret over hourly RVUs and wonder if AI can increase their productivity by 5 or 10 percent, AI is already busy saving lives in Africa.