Voice recognition: Optimization from a workflow and usability perspective
Images
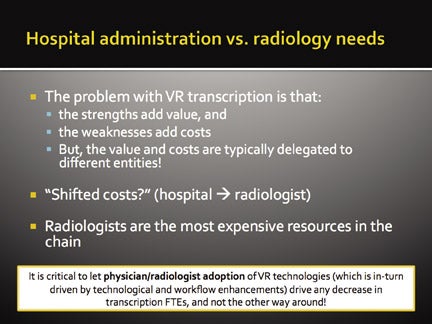
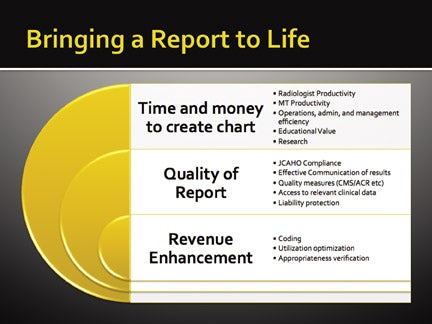
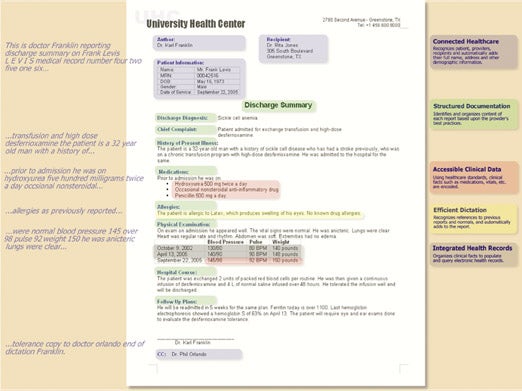
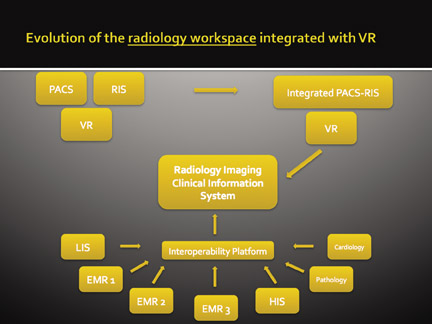
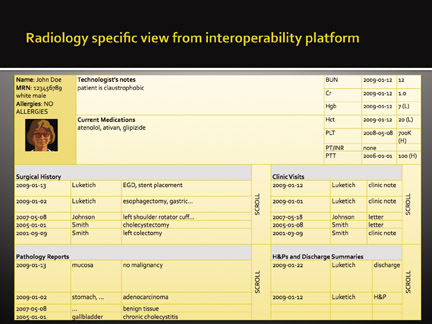
Dr. Shrestha is Medical Director for Digital Imaging Informatics, Chief, Division of Radiology Informatics, University of Pittsburgh Medical Center, Pittsburgh, PA.
I am primarily interested in how voice recognition (VR) and the technologies that surround it serve as a cohesive bond between all the different applications that now form our radiology workspace. Today, VR is an enabler of workflow optimization. The technology is certainly gaining a lot of respect in terms of its effects on productivity and its effects on the quality of service in radiology. However, we have all heard horror stories about how VR systems have affected, or not affected, certain productivity parameters in the past. That said, the technology is changing rapidly, and it is being incorporated into our workflow paradigms at a brisk pace. Today’s reporting systems are smarter,faster and we are seeing an increasing usage of VR technologies in electronic medical records (EMRs).
The technology is an enabler. It has traditionally been viewed as a means to offset the shortage of qualified transcriptionists, reduce overall costs of transcription and to decrease report turnaround time. These three factors have been the most important drivers in the adoption of VR, especially in academic institutions. I would contend that there is a fourth factor in that these tools support the increasing volume of medical reports that Dr. Siegel alluded to in his introduction. The technology also facilitates the increasingly geographical decentralization of care delivery, which is becoming commonplace across the country.
Second-level goals for VR
As these systems gain more clinical acceptance, we have identified several second-level goals. The second-level goals are increasing the accuracy of these systems, improving physician acceptance of both the technology and of the newer applications that we are developing around it, and improving the functionality of VR systems. In terms of functionality, we are moving past using VR to simply type with our tongues.
VR adoption differs quite a bit in academic vs. private radiology groups. Academia has the benefits of what we term “cheap labor.” Our residents and some fellows do a lot of the grunt work on top of what is necessary to make a VR solution work. Looking at the private-practice setting, some groups do not use VR because they are very happy with their efficient and dedicated transcription teams. In cases where those teams exist with minimal turnover, private groups are extremely happy with their services. Other private radiology groups consider VR to be placing the burden of transcription and editing on the radiologist, which they consider to be too valuable a resource to spend hours in that manner.
Some radiologists adopt VR because it has been thrust upon them. So now that they don’t really have a choice, they are now the new champions of VR. Some radiologists switched to VR from inferior transcription services. Those radiologists may have contended with delayed report turnaround times, numerous mistakes, and high turnover rates in the transcriptionist pool.
Challenges in a VR workflow
Part of the problem with VR transcription is that the strengths add value, but the weaknesses add cost to the entire healthcare system. Further, the value and the costs are typically delegated to different entities (Figure 1). Oftentimes, the needs of hospital administration are not the same as the needs of the radiology department. So if the contention is that the hospital wants to save money by eliminating transcription, should the end result be that the work is transferred to the radiologist? That is not the wisest approach, because radiologists are the most expensive resource in this chain. My recommendation has always been to let physician or radiologist adoption of VR drive the decrease in transcription FTEs; not the other way around. Physician adoption is a critical component for success and thus cost savings.
There are two main motivators that drive physician adoption: Leveraging current technology and providing a choice. There a variety of different needs within radiology. We have residents, fellows and attendings, all with different levels of skill and expertise. So we need to offer choices. And we actually have the technical capabilities, at this stage, to offer more choices to our radiologists.
We built our own in-house system at UPMC, and it is based on a speech engine from a company called M*Modal (Pittsburgh, PA). We designed it such that our focus is always on the images. We do not have text appearing on the screen, as we are transcribing our cases. And we have found this to be highly efficient. Our residents love the system and our attendings cannot disregard the system because of its workflow efficiencies. It is a PACS-driven workflow and VR technology is enabling much of this.
Radiology reporting essentials
I’ll touch briefly upon some of the radiology reporting documentation essentials. There are some strict parameters that the American College of Radiology (ACR) and other bodies are actually setting. We need to account for these essentials, such that we might be able to use the technologies to help us be more efficient.
The main concern is that if something is not dictated or documented, the service was not provided. So if we have done a 3-dimensional computed tomography angiography (CTA) procedure with postprocessing, and we have not documented this in the report, the procedure never happened. The ACR standard for communication is that we have to report the reason for the study. We also have to report everything that was done, for instance, we if we obtained plain radiography we have to state the number of views; and for magnetic resonance imaging (MRI) or CT we have to state the contrast used.
At this stage, simply using the VR technology to type with your tongue is limiting the true capabilities of VR. We are looking to maximize the capabilities of natural language processing in conjunction with the HL-7 Clinical Document Architecture (CDA) standard.
Some of the essentials we need to report are pertinent facts like history, outcomes and we need to provide evidence that the servicewas actually rendered. These are the parameters that absolutely have to be captured and completed, preferably before the report is signed off.
Next-generation transcription
So what does the next-generation transcription application really look like? We are in an era of distributed digital imaging. We’ve moved rapidly from analog to centralized analog, to centralized digital, where the proliferation of PACS was very rampant across the industry. And we are now moving towards an era of distributed digital. In this environment, the true value of a combined radiology workspace, enabled by VR technologies plays an important role.
We want to bring the report to life. It should not just be a collection of flat ASCII text. There are three aspects to this (Figure 2). One is the time and money required to create the chart. Consider things like radiologist and medical transcriptionist productivity and the efficiencies of that process. The quality of the report is also extremely important in terms of whether it is communicating the results out to the referring clinicians. And the third factor is revenue enhancement, in terms of coding, utilization optimization and appropriateness of verification.
Next-generation VR components
There is an increasing push towards standardization. Clinical decision support is one of the pillars of a next-generation VR platform. We also need better integration with modalities. If we are doing an ultrasound, and we are capturing various measurements, we should already have those measurements pre-populated in the report, such that even before you start the dictation, part of your report is already complete.
We are doing a lot of interesting things at UPMC in terms of interoperability between our radiology systems and the EMR. We want to bring this information to the radiologist’s workspace. This will enable the radiologist to get clinically relevant data points via the interoperability platform. That information can be incorporated directly into the report. We also need to incorporate structured reporting, natural language processing and CDA. Critical test result management is an important factor in all of this. We have the potential,with natural language processing for example, to really automate this process. And then lastly, but not least, we can better manage our practice with data analytics.
We must leverage natural language processing and CDA. Some examples would be automated clinical alerts; automated codification of findings within the report; and live alerts of possible alternatives based on CPT code. Imagine if that information could pop up as you are dictating a report. We could also integrate best-practice data from initially the hospital, then the hospital enterprise, and then other collaborating institutions.
And one idea that we are developing in our institution is a resident report-discrepancy portal. After residents dictate a report, many times attendings go back in, before the report is finalized, and make significant changes. Without a tool like this, the resident would have no way of knowing such changes were made unless he or she went back into the report to review the final. So if we can automate this process, and maybe have all of this data feed to a portal that the resident can then track over the months that he or she spends, say, in mammography or women’s imaging, that would be extremely valuable.
Standards
Information flow between radiologists and the EMR is now being enabled through a greater adherence to communication standards like those advocated by the RSNA, the Society for Imaging and Informatics in Medicine (SIIM), the DICOM Working Group 20, andHL-7 CDA. All of these groups are working towards standardization of the information flow. And this includes creating a standard channel for sharing the clinical details, in both structured reporting as well as in narrative radiology reports. However, the critical step in making this process work is improving the communication of structured documentation between the imaging systems and the clinical information system (CIS).
So this brings us to the possibility of using a conversational document as opposed to the stark contrast of just typing with our tongues, which is primarily what a lot of us are doing these days with VR. We have the potential to transform speech directly into structured clinical documents. In Figure 3 the radiologist is in a conversation mode, speaking out the report. As he is speaking the report in conversation, the natural language processing (NLP) engine at the back end of this is capturing pertinent information and putting it in the structured report. This is not science fiction. This is reality. And we are actually reading this way in a test environment as we validate the solutions with our industry partners.
This also raises the possibility of semantic interoperability. With this, we are able to solve the fundamental challenges with EMRs by allowing clinical decision support, alerts, data-mining, and many more features. We can enable the interoperability that is required and really improve the overall quality of clinical documentation.
Integrated radiology workspace
The move towards an integrated workspace is extremely important. The goals are to build straightforward interfaces, combined with uncomplicated and stable workflow orchestration. The idea here is not for us to cause more problems for the radiologist, but to really streamline the process. We need to improve the existing landscape of radiology communication, and this is extremely important in terms of re-engaging the clinicians and the surgeons back into the radiology workflow. Doing this will bring additional value into this paradigm.
In terms of the workspace, gone are the days of the PACS needing to integrate with the RIS and having the VR application in a separate silo. We are moving towards an integrated RIS/PACS platform with VR that’s somehow interfaced or integrated into that application. But even beyond that, we’re moving towards a radiology imaging clinical information system (CIS); one that lies on top of the interoperability platform that then communicates to various EMR platforms (Figure 4a), the laboratory information system, pathology, cardiology, etc., bringing out the true value of all of this clinically relevant data, pulled in front of the radiologist at the time of his read.
In Figure 4b, I present an example of the radiologist-specific view, from our interoperability platform. We can pull up relevant information that would be tremendously beneficial for the radiologist. We are also developing this with a multimedia report repository in mind. This methodology would capture key images, alongside the text of the report. The key images would be embedded as thumbnails in the reports.
We have to provide more than simply flat ASCII text files through an HL-7 interface into the RIS. If we can have this multimedia report repository, it would create a dynamic report, where the physician clicks on a condition or a symptom, and links directly to the reference of the related information. At the same time, there’s a multi-application integration directly into the repository. So if we click on a thumbnail of a 2-dimensional image, it launches our PACS. And if we click on a snapshot of the 3-dimensional image, it launches our thin-client 3-dimensional application. We can then postprocess the study and get additional data. We can also leverage the Web-based platforms and integration points back to the EMR, RIS, and any other systems through an interoperability platform.
Conclusions
Healthcare currently represents 85% of the global personal computer and server-based speech recognition market. Within radiology, VR technology holds the promise of being far more than just a tool to convert speech to text. And I think this has become clear with some of the examples that I mentioned earlier.
By incorporating the natural language processing tools, we truly have an opportunity to enable interoperability and provide appropriate clinician support, as part of the larger CIS system. Lastly, VR and related technologies, as well as evolving standards around them, are really enabling us to make rapid and paramount changes in the radiology workflow. The goal is no longer to optimize our silos of information but to create a cohesive radiology workspace, with VR technologies being the glue that holds all of these things together.
Discussion
ELIOT L. SIEGEL, MD: Rasu, that was really an excellent overview. And I’ve got to start with the most provocative topic that you talked about. In regards to this whole idea of conversational report generation, help me understand how this works. So the idea would be that I would have a “conversation“ where I would communicate the ideas in a manner perhaps similar to what I would do for a fellow that I had, and then expect that system to take those ideas and to generate a report or structure a report from those?
DR. SHRESTHA: That’s correct.
DR. SIEGEL: So the first question that I have is from a medical-legal perspective. What you’ve actually reported, and the nuances associated with it, may not be translated. But I guess the idea then would be that you would review the computer’s interpretation of it?
DR. SHRESTHA: That is right.
DR. SIEGEL: And would the computer have the ability to learn, as time went on, based on its interaction with you? So give us some details.
DR. SHRESTHA: Absolutely. So what we’re talking about is obviously a new technology that we’re playing around with, the capabilities of which we don’t fully understand, at this stage. But it’s really about moving from speech recognition to speech understanding. So that’s that concept.
And the idea behind this is to possibly use some of the natural language processing technologies, etc., that we have, to structure or better structure our reports to add more consistency to them. We want to improve the quality of our reports across a wide variance that we’re seeing in the department, from radiologists to fellows, to various levels of attendings. We want to have one unified, distinct look and communication style for our referring population. We want to have a united face that is of the highest quality. So that is the general idea behind this.
And as far as the medical-legal side of this is concerned, it is not as simple as speaking into the microphone in conversation mode, and then the computer sends out the report. You actually have the opportunity, and this is part of the workflow, to have the structured report presented to you. And the idea is that the software acts as more of an editor than the radiologist. So the radiologist is really only making minor corrections. The natural language processing technology structures the report in the manner that you have specified, and if there are any specific errors, a right-to-left discrepancy for example, it would then highlight that. Plus, at the same time, this gives the radiologist an opportunity to approve the report before completely signing off.
DANIEL L. RUBIN, MD, MS: So it sounds like a very fascinating and interesting functionality. One thing that seems that might be missing from it is a prompting to the radiologist of what the content is. If you are using a conversational model, you may be incomplete in your dictation. One thing that’s coming out of the Radiological society of North America (RSNA) structured reporting initiative is presenting a template for the radiologists, so they are aware of what should be in a report. I don’t know what your experience will be like with this conversational system, especially if radiologists using it are not aware of the full structure that they should report. They may leave things out, and then that will interrupt the workflow, and they need to put more time in.
DR. SHRESTHA: That’s a very good question. And, in fact, at this stage right now, we’re actually developing these templates and incorporating some of these templates in the technologies I talked about. So we have a place to start and a method of integration when the larger bodies like RSNA or ACR finalize their standards.
DR. SIEGEL: As we talk about the future, one of the things I’d really love to be able to do is not only have the speech engine be able to create reports for me, but actually to navigate and interact with the system. So I want the same intelligent speech system to be able to respond when I say “Show me all of the unread neuroradiology cases that are done on MRI.” Or if I ask the system to go to the next study, or display the next series, or show me the old study and the new study side by side, it should be able to recognize and respond to those commands. And so with the work that you’re doing, has there also been the potential to be able to have the same engine to perform navigation and information-retrieval tasks?
DR. SHRESTHA: Actually, yes. And that’s a fantastic question. Primarily all of the things that we talked about address the front-end side of things. But on the back-end, as well, we are going in and we are re-indexing. We have this project where recently we have re-indexed about 500,000 reports. These are reports in flat ASCII text files that we have re-indexed on the back end. And we then built an interface to a front-end graphical user interface (GUI) for us to intelligently query this index. And, within milliseconds, we can get exactly what a radiologist is looking for.
So what we are seeing is if we are forced to wait on vendors, especially if it goes through an HL-7 interface back to the radiology information system (RIS) or the EMR application, and we are waiting for them to adopt the CDA standards, it could take awhile. So a possible work-around to this is for us to re-index this database. We now have it as a live, running index on the back end. So when you are doing these specific queries in the radiology environment, possibly in the ED or in the clinical environment, you’re not actually tasking the production system; you’re actually tasking or querying this live, re-indexed database.
WILLIAM W. BOONN, MD: At our institution, we’ve been solely using voice recognition for more than 10 years now—so much so that a lot of our residents probably have never dictated a full report from scratch. There are a lot of staff who lament the fact that all the residents are really doing is filling in the blanks on a report. They are concerned that the residents aren’t synthesizing the report into a single impression. Do you see this as a problem, or do you think that this concept really is outdated, and that we really do need to move toward a more structured style of reporting.
DR. SHRESTHA: I don’t think it’s outdated. In fact, I think it’s a very real problem. And the way we are tackling it, at our institution, is that we actually don’t allow residents to use templates. They have to dictate from scratch. Many of my colleagues are adamant about this, and I’m definitely on their side. As far as the learning process is concerned, residents really have to learn the fundamentals first. As they progress through their medical education, maybe towards the third or final year of their residency, we would give them the ability to use the full toolset. You don’t want it to be the case where they are done with their training and then they go out into the real world, having used VR for 10 years, and not know how to report without it.
KHAN M. SIDDIQUI, MD: So, talking about reporting, a lot of radiology reporting is not complete just because the imaging study is completed. A lot of times we receive additional data that could alter the final report. From the perspective of a clinician who is trying to look for patient information in this convergent environment, what are the automated methods of bringing in data after the imaging study is complete, to supplement the report?
DR. SHRESTHA: That’s absolutely an important part of all of this. It’s common that we are done with our report, or we think we’re done with our report, and suddenly there is an additional piece of data that comes in that could, at times, really affect the findings. One of the ways that we’re trying to tackle this is by building upon the interoperability platform. We are creating live integration points between our EMR and the laboratory information system, pathology, cardiology systems, and many more. We are bringing this data through the radiology front-end portal, so the radiologist has all of the pertinent information at the point of care.
At the same time, we are also trying to push for what we’re calling imaging interoperability. So the first step in that is defining what imaging interoperability really is. Is it just about making sure that the text of the reports are able to interoperate with each other, and that they are presented in a common format, no matter which platforms they were generated in? Or is it also about potentially looking at pathology images, and radiology images, and having some of the synergies in terms of having these other fields really come together in one platform. The goal should be that when you are reporting you have all the information you could possibly need.