The Compounded Value of AI Beyond Radiology
By Heine L
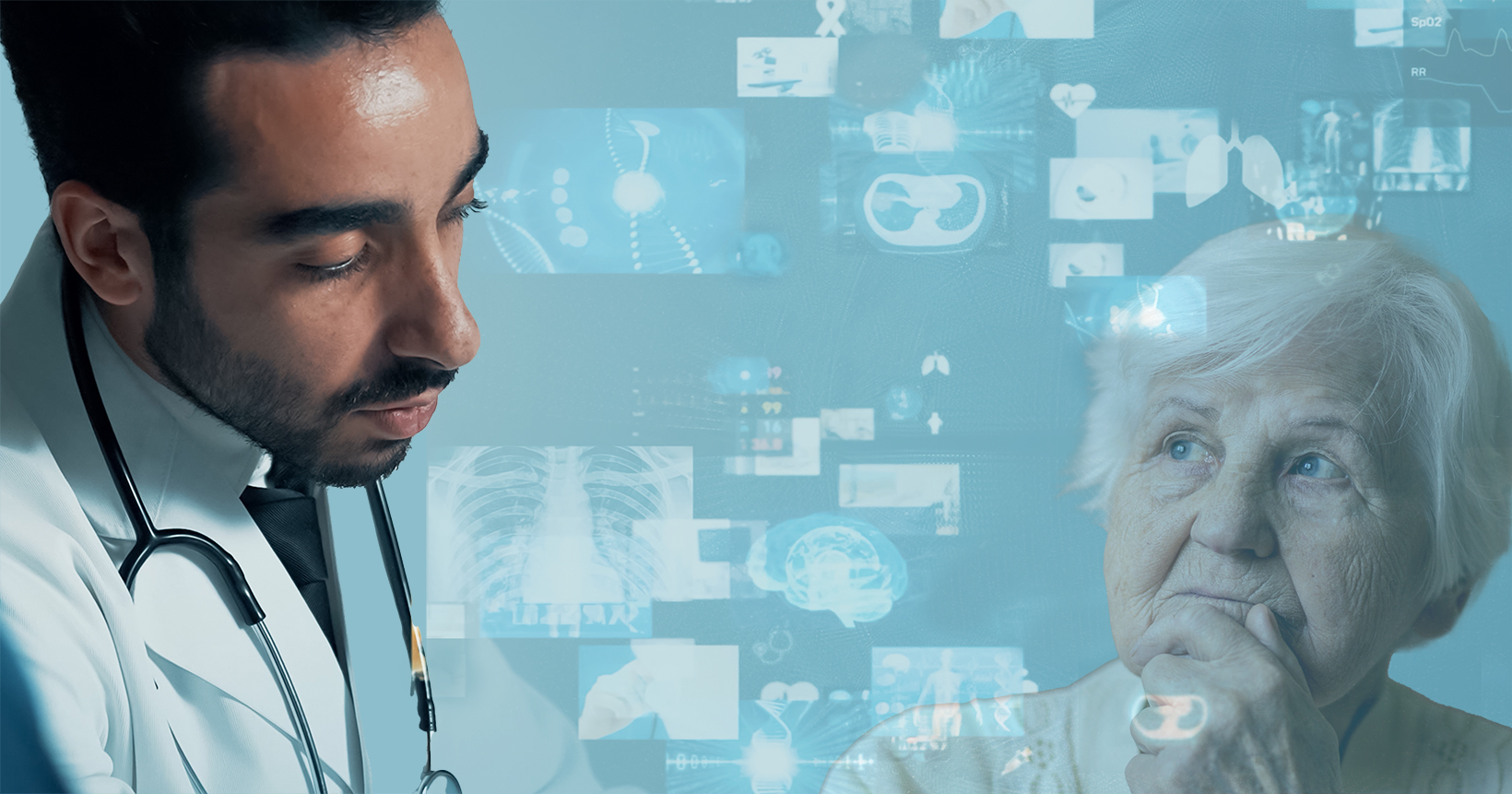
In healthcare, doctors help people; artificial intelligence (AI) helps doctors help people. It may sound trite, but it is important to adopt this philosophy as a guiding principle in product development. The US Food and Drug Administration (FDA) has approved close to 700 AI-enabled medical devices,1 and an even larger number of algorithms are being developed and deployed in research institutions. Each of these products and algorithms can handle a specific task, often with superhuman performance. However, it is easy to lose track of what is most important: the impact of these products on patient care.
Historically, AI products have faced several challenges to clinical implementation and, therefore, to realization of their full potential within the broader healthcare system. For one, an important bias that plagues AI algorithms relates to the heterogeneity of medical scans; depending on whether the software or algorithm being used was trained on them, different acquisition protocols or scan quality can cause unreliable results.2
Overfitting might also happen, causing the software to underperform on new sets of data. This can occur when certain types of data are under-represented in the training set. An example of this is a skin-cancer evaluation application trained on only one skin color.
Luckily, these issues are beginning to be addressed. The significance of racial bias in radiology3 is reflected in current guidelines,4 and some institutions are tackling the issues by providing open source data and labels. Large databases are available to innovators tackling cancer 5 and COVID,6 and more are expected in the coming years. For instance, the Oregon-Massachusetts Mammography Database project aims to catalogue 220,000 annotated mammograms with ground truth labels.7
Rigorous clinical evaluation of real-world use by independent third parties is the best way to thoroughly assess AI-enabled products and the possible biases affecting their performance. Studying accuracy, sensitivity, specificity, and user interaction with the AI solution is the first step in quantifying patient impact. Such assessments will also inform further software development, and thus improve the software and its value to patients.
The clinical usefulness and value of AI products along the entire patient pathway deserves more attention. Nowadays, evaluations of such tools and technologies are often limited to assessing their impacts on radiologist efficiency, accuracy,8 consistency of diagnostic evaluations,9 and/or cost.10 While these assessments are necessary, useful, and valuable, AI products could have compounding effects on other specialties. An important next step in AI development would be to reuse AI results within multiple steps of the healthcare pathway. For example, results from a screening AI could also be used for diagnosis, interventional therapy, measuring treatment response, or even clinical trials. The potential additional benefits of such compounding effects could positively impact AI utilization in healthcare as a whole.
Take prostate MRI. Current recommendations call for MR imaging prior to biopsy to decrease the number of biopsies and missed cancers.11 AI can assist radiologists,, especially trainees, in evaluating the prostate and in creating structured reports and segmentations.12
The software output can then be imported into fusion-capable biopsy systems. This would free up urologists’ time, as they no longer would have to manually segment the lesions based on textual or paper-drawn reporting. The same segmentations can also assist other physicians, for instance, by informing treatment-dose calculations to reduce damage to surrounding tissue, or in performing MRI-guided radiotherapy.
A clear understanding of the larger healthcare system around each AI product’s use-case will reveal what features can provide a more complete understanding of AI’s real value. Evaluating this is not an easy task, as the benefits will be different for each stake-holder. More research methods are needed to focus on compounded benefits, particularly for products whose costs are primarily borne by one department, while the benefits accrue across other service lines.
Artificial intelligence will bolster the performance of radiologists and that of the wider healthcare system. More real-world studies of diagnostic AI will demonstrate clinical utility for the radiology department and beyond. When we think more holistically about the positive effects on the overall healthcare system, we can truly assess the level of value a given product adds to patient care.
References
- FDA. Artificial Intelligence and Machine Learning (AI/ML)-Enabled Medical Devices. https://www.fda.gov/medical-devices/ software-medical-device-samd/artificial-intelligence-and-machine-learning-aiml-enabled-medical-devices (2023). Accessed December 11, 2023.
- D Drukker K, Chen W, Gichoya J, et al. Toward fairness in artificial intelligence for medical image analysis: identification and mitigation of potential biases in the roadmap from data collection to model deployment. J Med Imaging (Bellingham). 2023;10(6):061104. doi:10.1117/1.JMI.10.6.061104
- Gichoya J W, et al. AI recognition of patient race in medical imaging: a modelling study. Lancet Digit. Health. 2022; 4: e406–e414.
- FDA. Diversity Plans to Improve Enrollment of Participants From Underrepresented Racial and Ethnic Populations in Clinical Trials; Draft Guidance for Industry; Availability. https://www.fda.gov/regulatory-information/search-fda-guidance-documents/diversity-plans-improve-enrollment-participants-underrepresent- ed-racial-and-ethnic-populations (2022).
- The Cancer Imaging Archive (TCIA). https://imaging.cancer.gov/informatics/cancer_imaging_archive.htm. Accessed October 27, 2023.
- MIDRC. https://www.midrc.org. Accessed October 27, 2023.
- The Oregon-Massachusetts Mammography Database. https://mpsych.org/omama/.
- Martins Jarnalo C O, Linsen P V M, Blazís S P, van der Valk P H M, Dieckens D B M. Clinical evaluation of a deep-learning-based computer-aided detection system for the detection of pulmonary nodules in a large teaching hospital. Clin. Radiol. 2021; 76: 838–845.
- Lotter W, et al. Robust breast cancer detection in mammography and digital breast tomosynthesis using an annotation-efficient deep learning approach. Nat. Med. 2021; 27, 244–249.
- van Leeuwen K G, et al. Cost-effectiveness of artificial intelligence aided vessel occlusion detection in acute stroke: an early health technology assessment. Insights Imaging. 2021;12: 1-9.
- Wei J T, et al. Early detection of prostate cancer: AUA/SUO Guideline Part I: Prostate Cancer Screening. J. Urol. 2023; 210: 46–53.
- Faiella E, Vertulli D, Esperto F, et al. Quantib prostate compared to an expert radiologist for the diagnosis of prostate cancer on mpMRI: a sngle-center preliminary study. Tomography. 2022;8(4):2010-2019. Published 2022 Aug 13. doi:10.3390/tomography8040168