Study Evaluates Issues to Consider When Implementing Medical Imaging AI
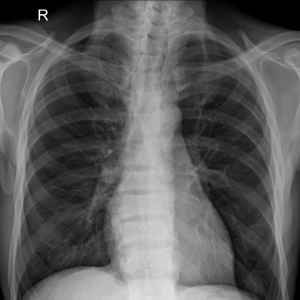
As AI is deployed in clinical centers across the US, one important consideration is to assure that models are fair and perform equally across patient groups and populations. To better understand the fairness of medical imaging AI, a team of researchers from Massachusetts Institute of Technology (MIT) and Emory University trained over 3,000 models spanning multiple model configurations, algorithms, and clinical tasks. Their analysis of these models reinforced some previous findings about bias in AI algorithms and uncovered new insights about deployment of models in diverse settings.
Previous work has shown that AI can predict self-reported race, gender, and age from chest x-ray images. In addition to underscoring these previous findings, the current work confirmed that AI can predict sex from ophthalmology images and illustrated that AI can predict age and gender from dermatology images, highlighting a potentially widespread issue across multiple different types of medical imaging modalities.
Why does this matter? If AI can predict demographic factors, it may use this information to inform its decisions. This is called a “heuristic shortcut,” where AI utilizes protected attributes like race or insurance status to drive its predictions instead of identifying and relying on pathological features in medical images. In this way, a model could make a prediction based on a demographic feature without any clinical basis, resulting in a model that has decreased performance among subgroups of people, potentially exacerbating existing disparities in the health care system.
Model performance tells us how well a model can accurately predict a condition or outcome, on average. Model fairness describes how well a model performs across different subgroups of patients.
For example, a model could be very good at predicting mortality from a chest x-ray, on average. But the model might be less accurate at predicting mortality when it’s applied to a younger patient compared with an older one.
“Model performance does not automatically translate to model fairness,” explained study author Judy Gichoya, MD, an associate professor in the Department of Radiology and Imaging Sciences at Emory University School of Medicine. “While high model performance is required for algorithm authorization, fairness is not always explicitly evaluated.”
While it was previously known that AI can predict demographic factors, and that heuristic shortcuts may lead to biased models, the researchers wanted to take a closer look at these relationships. They performed analyses to quantify how well a model could predict an attribute—known as the degree of encoding—without being given demographic information. Then they evaluated the performance of the model among different patients in that subgroup.
What they found was this: the stronger the encoding of a demographic factor, the worse the model was at being “fair” when performance was analyzed with respect to that factor. For example, when the researchers analyzed a subset of the radiology data, they found that the models could correctly predict a patient’s age approximately 75% of the time, meaning that age was strongly encoded. What’s more, these models did not perform equally across different age groups—in fact, they had a 30% fairness gap between elderly (ages 80-100) and young (ages 18-40) patients, meaning that the model had a 30%decreased performance between these two different age groups.
The researchers analyzed the degree of encoding of age, race, sex, and the intersection of race and sex in radiology models, finding that models could predict these attributes from chest x-rays with varying degrees of certainty. They also analyzed the degree of encoding of age and sex in dermatology and ophthalmology models, again finding that the models could predict these demographics from medical images.
Focusing on the radiology models, which had the most available data, the researchers observed the same trend—the better a model was at predicting a demographic factor, the less fair it was between patients spanning that demographic (young versus old, Black versus white, female versus male, for example).
“Our findings were very consistent: the more strongly the attribute is encoded, the larger the fairness gap is,” said first study author Yuzhe Yang, PhD, who performed this research as part of his doctoral work at MIT. “This correlation held true across every model that we evaluated, further reinforcing that AI models that rely on heuristic shortcuts to make their predictions have larger fairness disparities.”
To improve model fairness, the researchers applied a few different methods to their models that improved the balance of the dataset (so that the data is more reflective of the patient population) and that removed demographic information (thereby breaking heuristic shortcuts). They found that these techniques could improve model fairness without significantly sacrificing model performance.
However, the researchers found that optimizing for fairness alone can sometimes impact other model metrics, such as model precision or calibration, highlighting the need to balance model fairness with other factors.
“There’s always a tension between model performance and model fairness,” explained Gichoya. “Blindly optimizing for fairness could ultimately hinder the model’s utility, rendering it less reliable when it makes its prediction.”
The researchers found that their methods to optimize fairness were effective—if the model was evaluated using data from the same source that it was trained on. This phenomenon is called “local fairness.”
When the researchers deployed these locally optimal models on a dataset from a different source, they still had high performance. However, the models weren’t necessarily fair. This indicates that as the distribution of the data changes, it can potentially affect the model’s fairness.
“Our study found that local fairness doesn’t necessarily translate when models are deployed in a different setting,” said Yang. “This suggests that models that are developed and optimized for fairness in one hospital system may not be fair if they are deployed in a different hospital system or region. Our results underscore that models should undergo periodic updates and testing to ensure fairness is maintained over time, as data distributions and real-world contexts may evolve.”