Scientists Develop Method to Score Accuracy of AI-Generated Radiology Reports
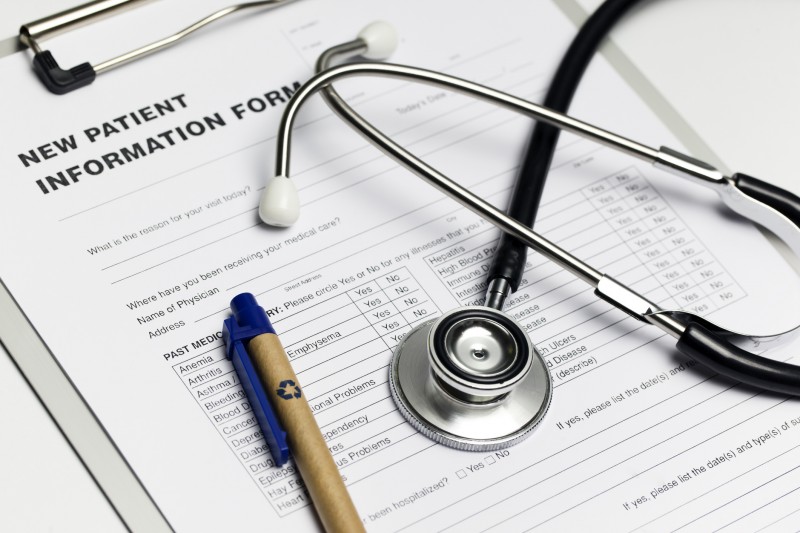
AI tools can quickly and accurately create detailed narrative reports of a patient’s CT scan or X-ray, greatly easing the workload of busy radiologists. These AI reports convey complex diagnostic information, detailed descriptions, nuanced findings, and appropriate degrees of uncertainty.
In addition to the availability of these tools, automated scoring systems that periodically assess these tools to help inform their development and augment their performance have also been released. So, how well do the current systems gauge an AI model’s radiology performance? According to a study published in Patterns by researchers at Harvard Medical School, the answer is good but not great.
Ensuring that scoring systems are reliable is critical for AI tools to continue to improve and for clinicians to trust them, the researchers said, but the metrics tested in the study failed to reliably identify clinical errors in the AI reports, some of them significant. The finding, the researchers said, highlights an urgent need for improvement and the importance of designing high-fidelity scoring systems that faithfully and accurately monitor tool performance.
The team tested various scoring metrics on AI-generated narrative reports. The researchers also asked six human radiologists to read the AI-generated reports.
The analysis showed that compared with human radiologists, automated scoring systems fared worse in their ability to evaluate the AI-generated reports. They misinterpreted and, in some cases, overlooked clinical errors made by the AI tool.
“Accurately evaluating AI systems is the critical first step toward generating radiology reports that are clinically useful and trustworthy,” said study senior author Pranav Rajpurkar, assistant professor of biomedical informatics in the Blavatnik Institute at HMS.
In an effort to design better scoring metrics, the team designed a new method (RadGraph F1) for evaluating the performance of AI tools that automatically generate radiology reports from medical images.
They also designed a composite evaluation tool (RadCliQ) that combines multiple metrics into a single score that better matches how a human radiologist would evaluate an AI model’s performance.
Using these new scoring tools to evaluate several state-of-the-art AI models, the researchers found a notable gap between the models’ actual score and the top possible score.
“Measuring progress is imperative for advancing AI in medicine to the next level,” said co-first author Feiyang ‘Kathy’ Yu, a research associate in the Rajpurkar lab. “Our quantitative analysis moves us closer to AI that augments radiologists to provide better patient care.”
Long term, the researchers’ vision is to build generalist medical AI models that perform a range of complex tasks, including the ability to solve problems never before encountered. Such systems, Rajpurkar said, could fluently converse with radiologists and physicians about medical images to assist in diagnosis and treatment decisions.
The team also aims to develop AI assistants that can explain and contextualize imaging findings directly to patients using everyday plain language.
“By aligning better with radiologists, our new metrics will accelerate development of AI that integrates seamlessly into the clinical workflow to improve patient care,” Rajpurkar said.