Routine CT Imaging Can Identify Diabetes Risk
Images
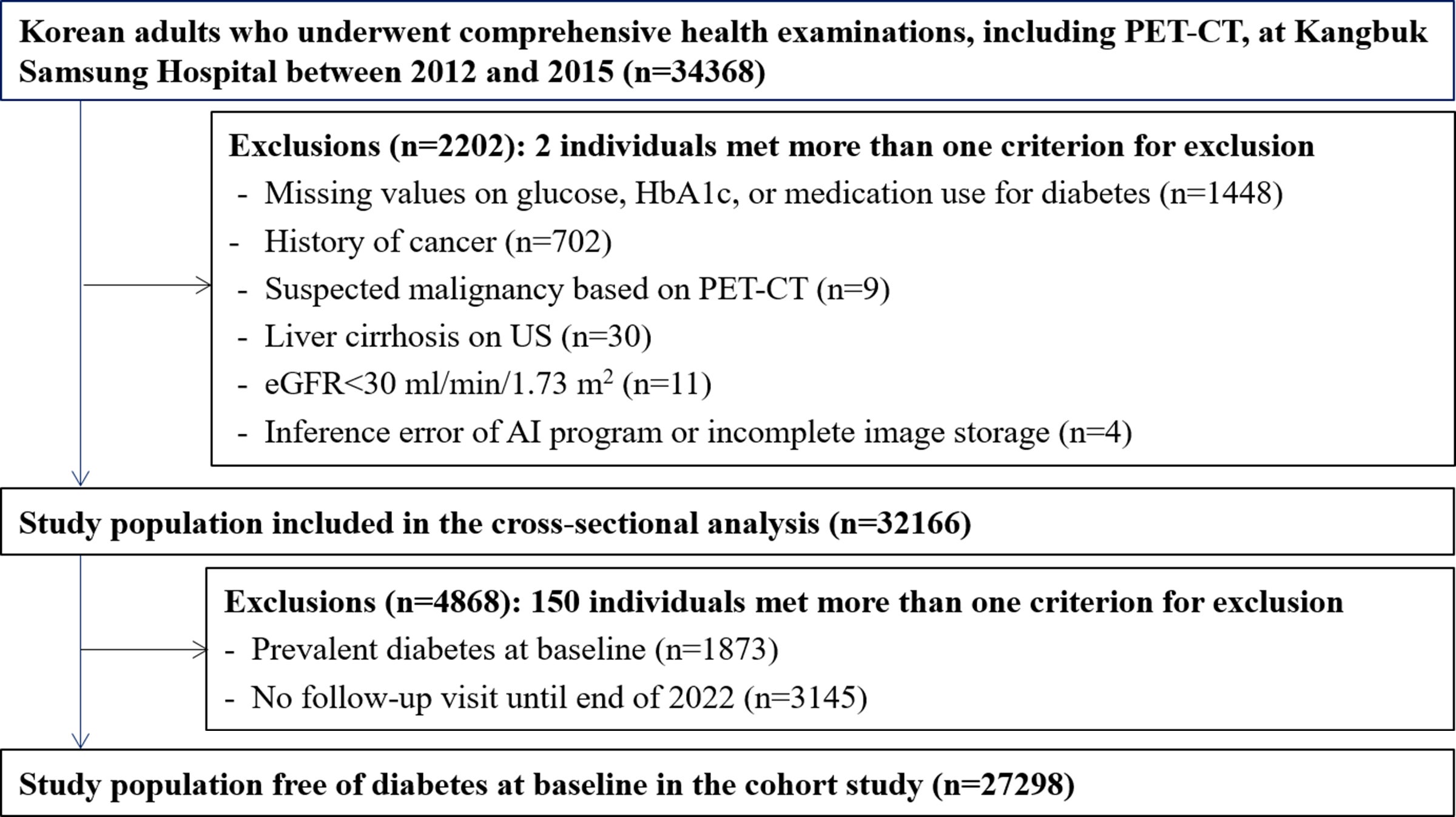
Automated multiorgan analysis of CT scans in people who undergo imaging for health screening purposes can identify individuals at risk of type 2 diabetes, according to a study published in Radiology. Researchers said the findings underscore CT’s value in opportunistic imaging—the use of information from routine imaging examinations to learn more about a patient’s overall health.
Research has shown promise for the opportunistic use of CT imaging beyond its primary clinical indication in incidental osteoporosis screening. CT performed for various clinical indications also has the potential to predict cardiometabolic diseases like type 2 diabetes mellitus, a common disorder that is frequently diagnosed late. However, the predictive ability of individual imaging parameters for cardiometabolic diseases remains underexplored.
For the new study, researchers in Korea evaluated the ability of automated CT-derived markers to predict diabetes and associated cardiometabolic comorbidities.
“Given the significant burden of diabetes and its complications, we aimed to explore whether automated and precise imaging analyses could enhance early detection and risk stratification beyond conventional methods,” said study senior author Seungho Ryu, MD, PhD, from the Kangbuk Samsung Hospital at Sungkyunkwan University School of Medicine in Seoul.
The study group included 32,166 adults ages 25 years or older who underwent health screening with 18F-fluorodeoxyglucose (18F-FDG) PET/CT.
Dr Ryu and colleagues used deep learning algorithms that have been clinically validated in numerous publications to analyze the CT images. The application of machine- and
deep-learning algorithms has made body composition analysis from images less labor intensive and less dependent on manual intervention. The algorithms enabled 3D segmentation and quantification of various body components such as visceral fat, subcutaneous fat, muscle mass, liver density and aortic calcium.
Diabetes prevalence was 6% at baseline and incidence was 9% during the 7.3-year median follow-up.
Automated multiorgan CT analysis identified individuals at high risk of diabetes and other cardiometabolic comorbidities. The index of visceral fat—the so-called belly fat under the muscles and around the organs of the abdomen—showed the highest predictive performance for prevalent and incident diabetes. Combining visceral fat, muscle area, liver fat fraction and aortic calcification improved predictive performance. CT-derived markers also identified ultrasound-diagnosed fatty liver, coronary artery calcium scores of more than 100, osteoporosis and age-related muscle loss called sarcopenia.
These markers outperformed traditional risk factors in predicting type 2 diabetes.
“The results are encouraging as they demonstrate the potential of expanding the role of CT imaging from conventional disease diagnosis to opportunistic proactive screening,” Dr Ryu said. “This automated CT analysis improves risk prediction and early intervention strategies for diabetes and related health issues.”
In the clinical setting, these CT-derived markers have the potential to improve the conventional approach to diabetes screening and risk assessment, Dr Ryu noted.
“By integrating these advanced imaging techniques into opportunistic health screenings, clinicians can identify individuals at high risk for diabetes and its complications more accurately and earlier than the current approach,” he said. “This could lead to more personalized and timely interventions, ultimately improving patient outcomes.”
The researchers plan to validate the findings in broader and more diverse populations to ensure the generalizability of the approach. Additional future research will explore the integration of CT-derived markers with other diagnostic tools and the development of predictive models that incorporate a wider range of health data.
“There is also interest in investigating the potential of CT-derived markers in predicting other metabolic and cardiovascular diseases beyond diabetes,” Dr Ryu said.