Research Suggests AI can Ask Another AI for Second Opinion in Medical Imaging
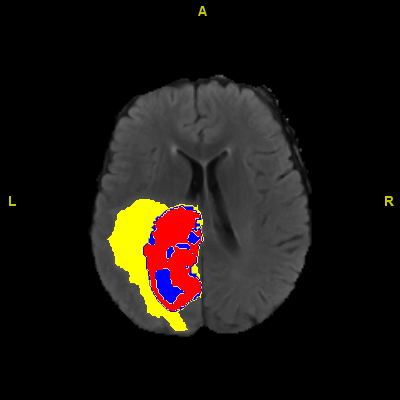
Researchers at Monash University in Melbourne, Australia have recently developed a co-training AI algorithm for medical imaging, aimed at emulating the process of seeking a second opinion. This advancement, published in Nature Machine Intelligence, addresses the scarcity of human annotated medical images by utilizing an innovative adversarial learning approach with unlabeled data.
The collaborative effort of Monash University's faculties of Engineering and IT holds great promise in advancing the field of medical image analysis for radiologists and healthcare professionals. Led by PhD candidate Himashi Peiris from the Faculty of Engineering, the research involved creating a "dual-view" AI system where two components compete in a unique manner.
The first part of the AI system emulates radiologists' expertise by labelling medical images, while the second part evaluates the quality of AI-generated labelled scans by comparing them against the limited annotated scans provided by human radiologists. This intelligent interplay between the two components enhances the AI's ability to provide accurate assessments, resembling the valuable process of obtaining a second opinion from a human expert.
In the conventional approach, medical experts, including radiologists, manually annotate medical scans by marking specific areas like tumors or lesions. These annotations serve as guidance for training AI models.
However, this method has drawbacks, relying on individual interpretations and leading to time-consuming processes, errors, and delays for patients awaiting treatment. Moreover, creating large-scale annotated medical image datasets demands substantial effort, time, and expertise.
To address these challenges, the researchers at Monash University have developed an innovative algorithm that enables multiple AI models to capitalize on the strengths of both labeled and unlabeled data. By learning from each other's predictions, the AI models enhance overall accuracy.
In extensive testing across three publicly accessible medical datasets, using just 10% labeled data, the algorithm demonstrated an average improvement of 3% compared to the latest state-of-the-art approach under identical conditions, as revealed by Ms. Peiris. This breakthrough promises to significantly improve medical image analysis and positively impact patient care.
The results achieved by our algorithm in semi-supervised learning are truly groundbreaking, surpassing the performance of previous state-of-the-art methods. What sets it apart is its ability to deliver exceptional performance even with limited annotations, eliminating the dependency on vast volumes of annotated data.
As a result, AI models powered by this algorithm can now make more informed decisions, validate their initial assessments, and provide more accurate diagnoses and treatment recommendations.
In the next phase of our research, we aim to extend the application of this algorithm to various types of medical images. Our goal is to develop a dedicated end-to-end product that radiologists can seamlessly incorporate into their practices. This innovative tool promises to revolutionize medical image analysis and significantly enhance healthcare outcomes for patients.