RAD-AID: Fostering Opportunities to Impact Global Health with Technology
Images
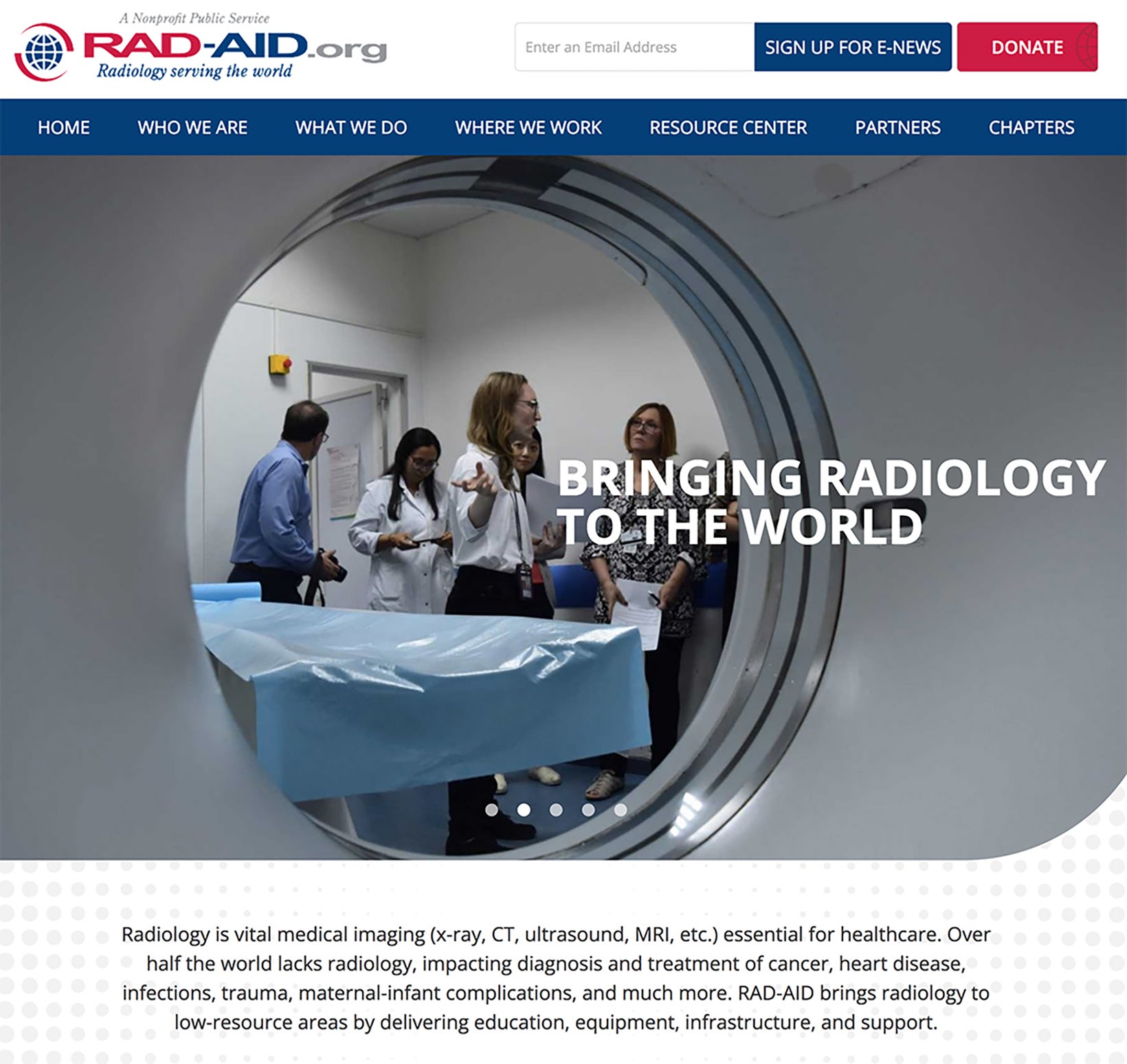
Since RAD-AID was founded in 2008, the organization’s vision has consistently focused on interdisciplinary approaches to increasing and improving radiology in low-resource communities around the world.
Indeed, RAD-AID builds radiology capabilities by assembling and integrating the perspectives of diverse radiology professionals such as radiologists, technologists, sonographers, residents, nuclear medicine imagers, nurses, informatics/information technology (IT) specialists, medical physicists, radiology administrators, and more.
In this debut of Global Health Imaging, we would like to focus on how we are leveraging artificial intelligence (AI) and other technology advances to improve global health outcomes through medical imaging.
AI and Medical Imaging
Radiology is never static; the specialty continues to grow and evolve with new technologies and scientific advances. One high-profile example of such advances is AI, which radiology is making ever-increasing use of to build software algorithms to assist clinicians in making healthcare decisions.
Initially, AI’s arrival on the landscape of medical imaging was perceived by many radiology professionals as a threat.1 It is becoming increasingly clear, however, that AI is serving more as an aid to medical imaging work rather than as a replacement for personnel. Medical imagers who understand this are in position to manage implementation of AI solutions that can dramatically improve health care.2,3,4
Where data privacy and medical device regulations have advanced frameworks, AI implementation will encounter a different set of steps compared to low-resource contexts where data privacy and medical device regulations are still in the early stages of formulation. Through its Teach-Try-Use model for AI deployment, RAD-AID is continuing to focus its efforts on ensuring that protecting patients, empowering health workers, and safeguarding privacy, are at the forefront of ethical priorities in the implementation of AI in radiology. 5
Remote Education
Other innovations include the use of advanced simulators that enable radiologists and technologists to practice real-time medical imaging procedures; RAD-AID is leveraging simulators particularly for interventional radiology education, where they can give trainees hands-on practice in angiographic procedures ahead of contact with patients. This kind of education can even be provided remotely through videoconference platforms to help provide low-resource regions with new avenues for skill development.
RAD-AID is using remote technology in teaching and case-based consultations. One example: the organization is developing a tele-ultrasound program, whereby the student is physically present with the patient while their teacher, consultant, or supervisor provides on-screen instruction from another location in real time. RAD-AID plans to apply the tele-ultrasound educational model to general body imaging, pediatrics, and midwifery/skilled birth attendant education.
RAD-AID is also working with many picture archiving and communication system (PACS) vendors to acquire and implement PACS and data-storage capabilities in low-resource institutions through the RAD-AID Friendship Cloud. The organization then provides a real-time, active platform for consultation and teaching, including remote radiology residency training on real cases. 6,7 The field of “implementation science” plays a major role in making it possible to deploy technology to develop strong educational impact. 3,8,9
Finally, technology is changing how global health specialists analyze health care disparities and resource allocation. RAD-AID, for example, is leveraging a geographic information systems (GIS) program to analyze health outcomes, economic scarcity, infrastructure, topography, and other data by fusing maps to produce advanced visualization of healthcare shortages and deficiencies. Geographic information systems can help inform the design of solutions to reach more people in need. Indeed, RAD-AID is using GIS to visualize geographic and population-based distribution of radiology resources to determine how they can be more equitably utilized across populations and regions. 10
In conclusion, this exciting spectrum of technology innovation comprises just one aspect of the multidisciplinary work of RAD-AID, whose aim and goals are to present medical imaging professionals with balanced and integrated strategies to help address healthcare disparities around the world.
References
- Langlotz CP. Will artificial intelligence replace radiologists? Radiology: Artificial Intelligence. 2019;1(3).
- Safdar NM, Banja JD, Meltzer CC. Ethical considerations in artificial intelligence. Eur J Radiol. 2020;122:108768. Epub 2019/12/02. doi: 10.1016/j.ejrad.2019.108768. PubMed PMID: 31786504
- Mahajan V, Venugopal VK, Murugavel M, Mahajan H. The Algorithmic Audit: Working with Vendors to Validate Radiology-AI Algorithms-How We Do It. Acad Radiol. 2020;27(1):132-5. Epub 2019/12/11. doi: 10.1016/j.acra.2019.09.009. PubMed PMID: 31818381
- London AJ. Artificial intelligence and black-box medical decisions: accuracy versus explainability. Hastings Cent Rep. 2019;49(1):15-21. Epub 2019/02/23. doi: 10.1002/hast.973. PubMed PMID: 30790315
- Mollura DJ, Culp MP, Pollack E, Battino G, Scheel JR, Mango VL, et al. Artificial intelligence in low- and middle-income countries: innovating global health radiology. Radiology. 2020;297(3):513-20. Epub 2020/10/07. doi: 10.1148/radiol.2020201434. PubMed PMID: 33021895
- Elahi A, Dako F, Zember J, Ojetayo B, Gerus DA, Schweitzer A, et al. Overcoming challenges for successful PACS installation in low-resource regions: our experience in Nigeria. J Digit Imaging. 2020;33(4):996-1001. Epub 2020/06/05. doi: 10.1007/s10278-020-00352-y. PubMed PMID: 32495127; PubMed Central PMCID: PMCPMC7522157
- Sedora Roman NI, Bochnakova T. Radiology abroad: a global health initiative in Guyana. Acad Radiol. 2017;24(6):753-4. Epub 2017/04/04. doi: 10.1016/j.acra.2016.09.027. PubMed PMID: 28366655
- Langlotz CP, Allen B, Erickson BJ, Kalpathy-Cramer J, Bigelow K, Cook TS, et al. A roadmap for foundational research on artificial intelligence in medical imaging: from the 2018 NIH/RSNA/ACR/The Academy Workshop. Radiology. 2019;291(3):781-91. Epub 2019/04/17. doi: 10.1148/radiol.2019190613. PubMed PMID: 30990384; PubMed Central PMCID: PMCPMC6542624
- Hosny A, Aerts H. Artificial intelligence for global health. Science. 2019;366(6468):955-6. Epub 2019/11/23. doi: 10.1126/science.aay5189. PubMed PMID: 31753987
- Daniels MJ, Game A, Mollura DJ, England RW. Strategic radiology outreach planning for underserved populations using geographic information systems. J Am Coll Radiol. 2021;18(4):537-44. Epub 2020/11/03. doi: 10.1016/j.jacr.2020.08.022. PubMed PMID: 33137295
References
Citation
DJ M, AM L,. RAD-AID: Fostering Opportunities to Impact Global Health with Technology. Appl Radiol. 2021;(6):36-37.
November 6, 2021