Quantitative Volumetry is Revolutionizing Neuroimaging
Images
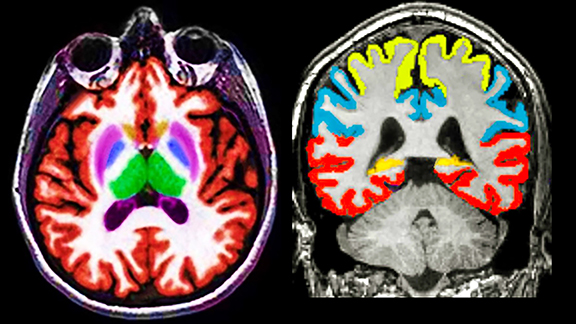
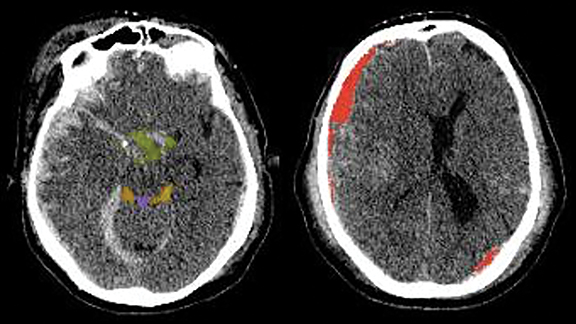
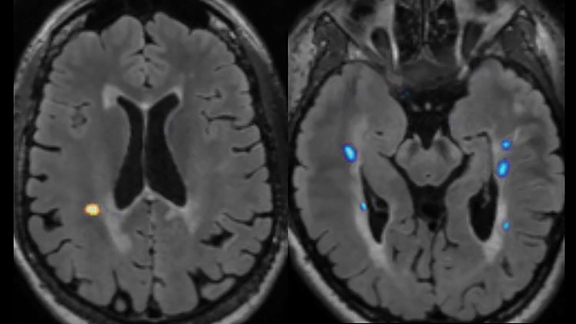
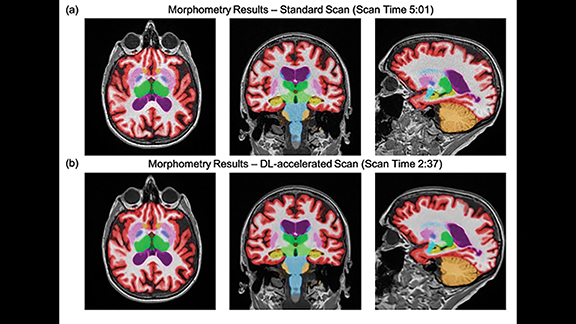
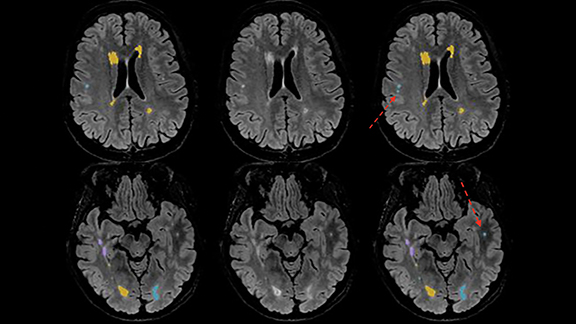
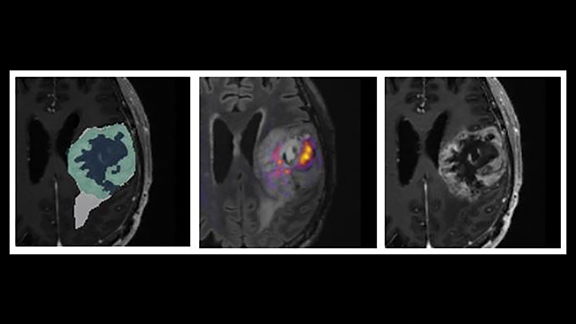
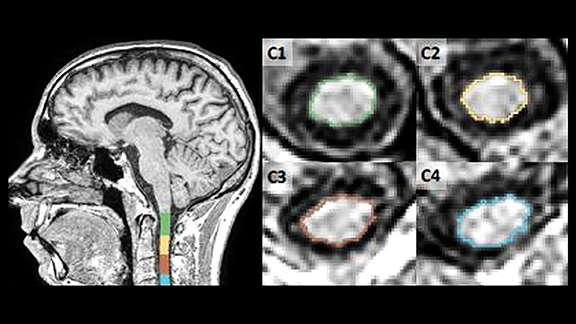
Artificial intelligence (AI) is impacting medical imaging in many ways, including enhancing value through quantitative volumetric neuroimaging (QNI). QNI offers gains in efficiency, accuracy, clinical relevance, reduced subjectivity, and even a modest return on investment and the potential to increase referrals.
Clinical Applications/Technique
While volumetric post-processing is useful in CT and PET, it is especially suited to the high spatial and contrast resolution of MRI, particularly in neuroradiological applications. Indeed, volumetric analysis is being applied to MRI of patients being evaluated for such indications as dementia, multiple sclerosis (MS), epilepsy, traumatic brain injury, and brain development. Volumetric analysis is particularly adept at tracking disease progression longitudinally.
Images are pushed to a cloud-based vendor portal with results delivered to PACS within 20 minutes of image acquisition (Figure 1). Quantitative analysis is most often applied to 3D T1 and FLAIR MR acquisitions and processing typically includes color-coded segmentation and volumetric assessment of anatomic substructures with comparison to a large normative age and gender-matched database. Values that fall more than 2 standard deviations from the mean are highlighted.
Brain CT applications focus on acute traumatic brain injury and stroke (icobrain) with additional benefits including sophisticated registration of serial data sets (Cortechs.ai’s CT CoPilot™) which speed interpretation (Figure 2). PET-CT applications are also being developed for dementia screening (Cortechs.ai’s PETQuant™).
Increases Efficiency
At a time when the focus is on relative value units, radiologists are under constant pressure to turn around studies quickly. Manually measuring tumors, subdural hematomas, hydrocephalus, disc extrusions, and other pathologies is laborious, time-consuming, and difficult to perform accurately. Longitudinal study evaluations are particularly challenging, owing to variations in head angulation and imaging protocols.
Machine-driven volumetric post-processing obviates the need for manual measurements. Automation boosts productivity and opens opportunities for the radiologist to spend more time on higher-level cognitive assessment. However, to benefit, radiologists must be familiar with these tools and trust their output.
The workflow impact of QNI tools was addressed in a small clinical trial where an experienced neuroradiologist was asked to dictate 50 MRI scans of 25 patients with MS. The scans, which were performed at 11 imaging centers, were presented in random order, with timed radiological evaluation performed twice for each patient, once without quantitative post-processing and once with the post-processing (icobrain). Productivity increased 60% with quantitative post-processing and a pre-populated summary report over evaluation without post-processing.1
SmartSoft is developing a convolutional neural network-based machine-learning (ML) analysis tool designed to improve efficiency for radiologists. CoLumbo, its CE- marked, FDA-pending tool, provides volumetric analysis of vertebral bodies, discs, the spinal canal, facet joints, neural foramina, and other spinal structures, and then automates and standardizes routine degenerative spine interpretation with a prepopulated report. According to an internal survey, SmartSoft estimates that radiologists spend approximately 17 minutes interpreting non-complicated lumbar spine MRIs and about 30 minutes interpreting more complex cases, yet they have fewer than 14 minutes on average per case for their routine clinical case load, a discordance that contributes to 30% omissions and errors.
Improves Diagnostic Accuracy and Clinical Relevance
The clinical value of imaging studies is largely contingent on the accuracy and impact of radiology reports. Accurate volumetric measurements can be critical for treatment. For example, identifying growth of a subdural hematoma can impact patient management, but detecting changes in size can be challenging, owing to the difficulty of manually obtaining accurate serial measurements across longitudinal exams, particularly given the concavity of the inner calvarial table.
Every neuroradiologist has interpreted contrast-enhanced brain MRI scans to longitudinally track a brain tumor, such as a complex skull base meningioma, where serial report impressions describe stability in tumor size. Tumor growth may only be evident when current scans are compared to serial exams over many time points, something that is time consuming to perform and may not always be done. Detection of subtle changes in lesion size is challenged by variability in acquisition parameters and head angulation between studies. AI-based analysis efficiently assesses and registers all prior exams, improving sensitivity to even the most slowly evolving lesions.
These same challenges are encountered when serially tracking lesion burden and brain atrophy in MS patients, as visual estimates of lesion change and brain volume are often inaccurate. Quantitative post-processing easily highlights evolution of disease by segmenting, color-coding, and quantifying baseline and dynamic (ie, new, enlarging, shrinking) plaques (Figure 3).
Plaque volume, whole brain volume, and cortical gray matter volume are known biomarkers of MS patient prognosis, with progressive brain volume loss and plaque progression portending worse Expanded Disability Status Scale (EDSS) longitudinal clinical outcome scores compared to MS patients with stable volumetric biomarkers.2 Definitive establishment of true disease progression could impact the neurologist’s decision to alter disease-modifying drug treatment.
Quantitative assessment may upgrade disease status. One study reports 24% active (enlarging or new) plaques and/or progressive brain atrophy without post-processing software versus 76% active plaques and/or progressive brain atrophy with the addition of automated volumetric analysis in the same patient cohort.1
Similarly, in epilepsy, detecting developing mesial temporal sclerosis via subtle asymmetry in hippocampal volumes can be difficult, owing to differences in head angulation. In the elderly, distinguishing normal age-related atrophy from a true emerging neurodementia syndrome can be difficult when relying solely on visual assessment of mesial temporal volume loss, especially over longitudinal studies.
Reduces Subjectivity
Referrers have high expectations for and must be able to trust the results of imaging studies. One of the primary goals of quantitative AI-driven tools is to reduce subjectivity and variability of reports. Differing interpretations of the same finding are problematic, and radiologists have begun to recognize the need for greater inter-reader, and even intra-reader, consistency in grading and quantitation.
Manual lesion segmentation of volumes suffers from high intra- and interobserver variability. Patient management is entirely different if one radiologist describes ventriculomegaly due to central atrophy and another attributes it to normal pressure hydrocephalus. Tracking subtle changes in ventricular size over serial exams can be difficult, but the task is made faster, more consistent, and more accurate with AI-driven tools.
Return on Investment and Other Concerns
Referring specialists who request QNI tend to rely upon it for follow-up exams, most often for patients with MS and dementia. Practices that offer these tools can distinguish themselves as high level centers of excellence. However, imaging practices may have concerns about costs and return on investment (ROI) when offering QNI.
Contracts with third-party vendors vary but are typically volume-based, annual subscription agreements. Fortunately, reimbursements generally track with add-on costs, providing a modest positive ROI. In circumstances with payor non-reimbursement, an appropriate front office patient pass-through charge is typical.
Data integrity remains a primary concern with respect to QNI, with known diminished accuracy when slices thicker than the preferred 1 mm collimation, or when 2D rather than 3D source images are employed. In one study, 3D FLAIR acquisition detected 42% more MS plaques than 2D FLAIR acquisition.3
Inter-scan protocol variability also undermines the value of longitudinal exams performed to assess disease progression. Variability between scanners is also a concern and longitudinal exams should ideally be performed on the same magnet, something not always practical in large imaging enterprises. Icometrix is attempting to address this concern in its ensemble AI models by applying a similarity index, data augmentation, and adversarial networks to make their algorithms more tolerant of MRI signal intensity differences.
Preliminary work compared biomarkers across multiple scanners and found good inter-scanner concordance of the quantitative values. Physics and ML meanwhile are used extensively in the Cortechs.ai NeuroQuantTM product line for modeling and correcting image artifacts, as well as for predicting and correcting contrast changes across scanners and protocols to maximize inter-scanner and scan/rescan consistency.
Concerns have also been raised about the reliability and consistency of quantitative biomarker data between exams. These concerns have been addressed in a recent prospective, multicenter, multireader trial that combined two different AI solutions to evaluate their impact on both image quality and quantitative image analysis (NeuroQuant™) consistency of 60% accelerated volumetric MRI scans processed with a commercially available, deep-learning (DL) tool (SubtleMR™) compared to that of standard of care (SOC).4
The trial found no difference in quantitative volumetric biomarkers or clinical classification between the accelerated DL-enhanced and SOC datasets. DL reconstruction allowed 60% scan-time reduction while maintaining high volumetric quantification accuracy, consistent clinical classification, and perceived superior image quality compared with SOC. This trial supported the reliability, efficiency, and utility of DL-based enhancement in quantitative imaging. Shorter scan times have the potential to boost utilization of volumetric quantitative MRI in routine clinical settings (Figure 4).
When present, segmentation errors (false positives and false negatives) can hinder radiologist confidence. Infratentorial MS plaques are often more conspicuous on T2W sequences, but current QNI tools analyze only FLAIR and T1W sequences. Image quality challenges also exist for many 3D FLAIR techniques and users encounter occasional false negative errors, particularly in the posterior fossa.
As with all AI solutions, translating a tool into an accurate and clinically beneficial product is a work in progress. New and improved software versions are continually emerging to solve the challenges encountered by their predecessors.
Initially, QNI tools employed rules-based algorithms with segmentation boosted by ML. Recently, DL-based techniques have shown promise in improving accuracy and sensitivity. Icometrix employs an ensemble approach to MS plaque segmentation. Deep learning is used to improve plaque segmentation in the posterior fossa and juxtacortical regions, while ML is still used to segment periventricular and deep white matter plaques, as ML does an excellent job of “plaque filling” in these regions, which often contain larger plaques.
Icometrix is also currently evaluating an additional AI layer that learns from the existing ML and DL analysis and serves as a “second-look.” The method considers candidates from both methods (ML and DL) and intelligently adds and removes to obtain optimal segmentation accuracy (Figure 5). Preliminary results suggest the highest inter- and intra-rater concordance was achieved with utilization of the third layer, with intra-rater variability equivalent to that of an expert reader.
Emerging Developments
Novel, AI-enhanced, quantitative tools are advancing in the marketspace. One promising emerging advance is CorTechs’ DL-based FDA-pending OnQ™ Neuro product for tumor segmentation and characterization (Figure 6).
Quantification of spinal cord atrophy is an innovative capability in development by icometrix leveraging sagittally acquired brain and spinal scans. On brain scans, the cross-sectional area at C2-C3 is a reliable marker for MS disease progression and for differential diagnosis of MS subtypes. This fully automated pipeline for quantification of spinal cord atrophy uses a combination of spinal-cord-toolbox (SCT) methods and customized development to improve the accuracy and robustness of spinal cord segmentation and labeling. The mean upper cervical cord area (MUCCA) values are important biomarkers of patient disability status in MS and can be determined using this fully automated software (Figure 7).
In summary, quantitative volumetric post-processing adds value to neuroradiology and integrates seamlessly into radiologic workflow. Its popularity has grown commensurately with progressive awareness of its benefits and successful adoption of AI strategies to overcome inherent challenges, such as using an ensemble approach (ML + DL) to optimize segmentation accuracy.
Quantitative analysis offers advantages to the patient, radiologist, referrer, and imaging facility. We anticipate widespread clinical acceptance of this technology as products and clinical indications continue to broaden, refine, and improve.
References
- Sima D, Wilms G, Vyvere TV. Icobrain’s Prepopulated Reporting Template for Multiple Sclerosis Follow-up. In: Proceedings of NeuroRAD Congress 2019, Frankfurt, Germany 2019.
- Van Hecke W, Sima D, Billiet T, et al. Short-term MRI-based Prediction of Clinical MS Progression in a Real World Setting (P5.2-067). Neurology 2019; 92(15): P5.2-067.
- Patzig M, Burke M, Bruckmann H, Fesl G. Comparison of 3D Cube FLAIR with 2D FLAIR for Multiple Sclerosis Imaging at 3 Tesla. Neuroradiology 2014;186: 484-488. DOI http://dx.doi.org/ 10.1055/s-0033-1355896.
- Bash S, Wang L, Airriess C, et al. Deep Learning Enables 60% Accelerated Volumetric Brain MRI While Preserving Quantitative Performance – A Prospective, Multicenter, Multireader Trial. In: Proceedings of ISMRM 2020, ASNR 2020, ECR 2021 and submitted for publication.
Citation
S B, LN T,. Quantitative Volumetry is Revolutionizing Neuroimaging. Appl Radiol. 2021;(3):38-42.
May 4, 2021