Multiparametric MR Radiomics Not Optimal for Differentiating HER2 Breast Cancer
Images
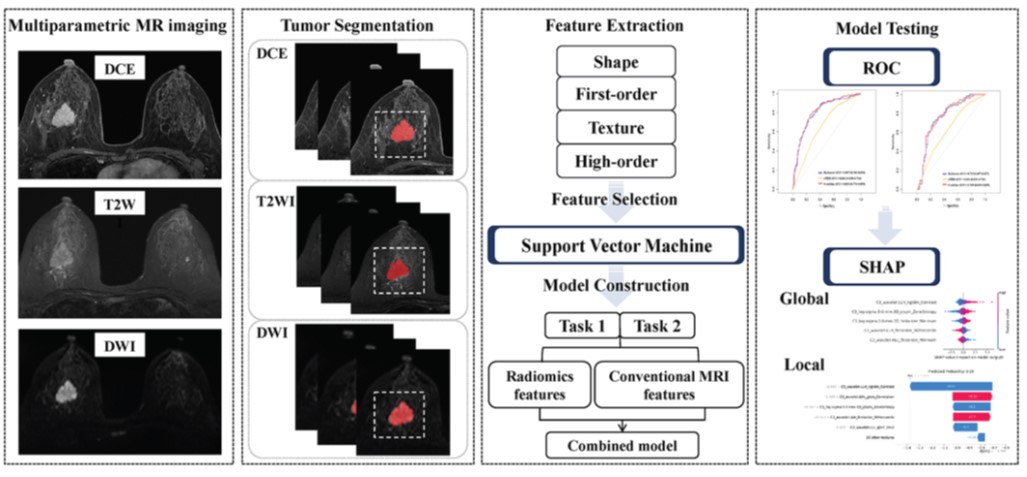
Findings from a study published in the American Journal of Roentgenology (AJR) indicate suboptimal performance of multiparametric MRI (mpMRI) machine learning radiomic models for noninvasive characterization of breast cancer with three-tiered HER2-expression status: HER2-zero, HER2-low, or HER2-positive.
“The study provides an example of the use of SHapley Additive exPlanation (SHAP) interpretation analysis to better understand predictions of imaging-based machine learning models,” wrote corresponding author Yuan Guo, MD, PhD, from the radiology department at Guangzhou First People’s Hospital in Guangdong, China. “The visual presentations from global and local SHAP analyses may provide a template for presenting complex data from future radiomics investigations in an accessible format.”
Guo et al. included 737 patients (mean age, 54.1 years) with breast cancer from two centers (center 1: n=578; center 2: n=159), who underwent breast MRI and had HER2 expression determined after excisional biopsy. Analysis entailed two tasks: differentiating HER2-negative (i.e., HER2-zero or HER2-low) from HER2-positive tumors (task 1), and differentiating HER2-zero from HER2-low tumors (task 2). For each task, patients from center 1 were randomly assigned in 7:3 ratio to training (task 1: n=405; task 2: n=284) or internal test (task 1: n=173; task 2: n=122) sets; those from center 2 formed an external test set (task 1: n=159; task 2: n=105). Radiomics features were extracted from early-phase dynamic contrast-enhanced images (DCE), T2-weighted images (T2WI), and DWI.
Ultimately, in external testing, mpMRI radiomics score provided AUC for differentiating HER2-negative from HER2-positive tumors of 0.757, and HER2-zero from HER2-low tumors of 0.754. SHAP analysis of model interpretations identified the strongest influence for early-phase DCE features for both tasks; T2WI features also had a prominent role for this second task.