Features neither an application nor a company make
By Tanenbaum LN
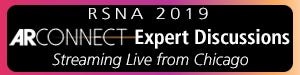
The market for applications based on artificial intelligence (AI) is heating up throughout health care, with an ever-growing number of offerings for diagnostic imaging. Numerous products have received FDA marketing clearance and CE marking in recent years. Many, if not all, to date are for narrow indications and, from the perspective of a clinical manager, are best described as features rather than products.
While the much-heralded ability to assess pediatric bone age from skeletal radiographs, for example, could be marketable as a freestanding product, the targeted market is small. Imagen has received FDA approval for wrist fracture, but the company won’t have a genuinely marketable product until it transfers learning and gains approval for broader, full skeletal applications.
Mammography, with its ever increasing study volume and complexity, as well as a shortage of qualified readers, represents a huge opportunity for AI. Curemetrix has regulatory approval for CMAssist, which can discern positive cases from a stack of screening mammography studies, but on its own, the practical impact of this capability is small. Again, the company will not have a truly complete offering until it gains approval for the full and functional computer-aided detection (CAD) capabilities that published research suggests can improve breast cancer diagnosis and clinical workflow, owing to fewer flagged false positives compared to conventional mammography offerings.
Many companies are attempting AI-based identification in chest X-rays of what computer scientists call anomalies, such as pneumothorax, pneumonia, and misplaced lines and tubes. Multiple triage-based CT tools (some of which are still under development) detect hemorrhage and stroke (eg, products from AIDOC, MaxQ-AI, Viz AI), spine fractures, intra-abdominal free air, and pulmonary embolism (AIDOC). To achieve broad relevance and adoption, however, these offerings must expand to encompass the full spectrum of entities that radiologists are required to identify or exclude—all perceptible imaging findings—and assess their pertinence to the patient’s condition and clinical concern.
The capabilities of all-encompassing stroke-based products must include detecting large-vessel occlusion, assigning ASPECTS scores, identifying bleeding, and mobilizing the treatment team via secure text or email. A complete product would further leverage the ability of machine language (ML) to synthesize multimodal information, including advanced studies like CT angiography and perfusion imaging, with patient phenotype, and to suggest the next treatment step based on predicted outcome. Once validated, the complete offering would be essential for suspected stroke patient studies. Individual features that don’t meet full exam requirements could still find a home consolidated into another product, or perhaps on the imaging device itself; eg, a portable X-ray unit could possess the embedded capability of notifying the technologist immediately of a misplaced tube, and a CT scanner could alert the technologist to urgent findings before the patient leaves the imaging suite.
A product that could set aside, or identify, “normal” findings on X-ray or CT exams would have immediate and universal appeal, especially in regions where personnel shortages prevent timely interpretation, and could serve to redirect resources to exams with a likelihood of positive findings.
Machine learning lies behind the enhancements in commercial offerings for quantitative MR- and CT-based neuroimaging. Initial products attacked the diagnosis of dementia (Coretechs’ NeuroQuant) and demyelinating disease (icometrix’ icoMS), an important but narrow range of diseases. Diversification was inevitable; both tools now cover both conditions and incorporate features to assess epilepsy and traumatic brain injury. Ultimately, to meet the requirements of a full neuro suite and attract customers, these companies will likely need to expand and diversify their products to encompass the range of neuroimaging analysis, including perfusion, and perhaps fMRI via organic development or alliances with other companies.
Fledgling companies and investors in the AI space should keep these concepts in mind. Just as a feature does not make a product, a narrow application doesn’t make a company. Those who eventually provide a suite of applications that can fulfill most, if not all, of the requirements of end users (broad triage of urgent findings on a class of exams, disease-based synthesis of multimodal information, and full indication-based assisted diagnosis) have the best chance of thriving in this rapidly evolving field.
Dr. Tanenbaum is Chief Technology Officer, Director of Advanced Imaging, Vice President and Medical Director Eastern Region, RadNet Inc.