Exciting Times for Liver Imaging
By Kelly M
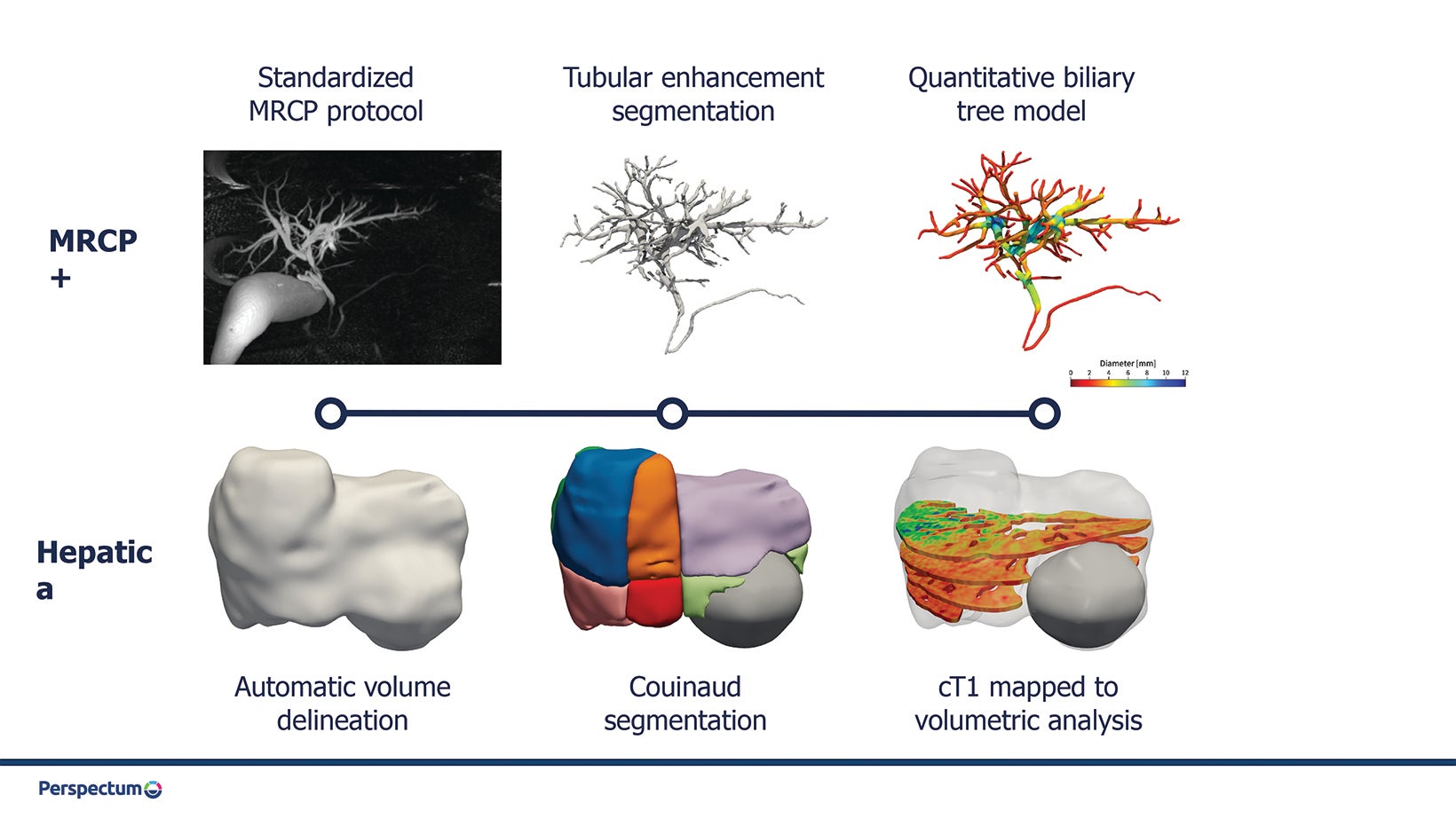
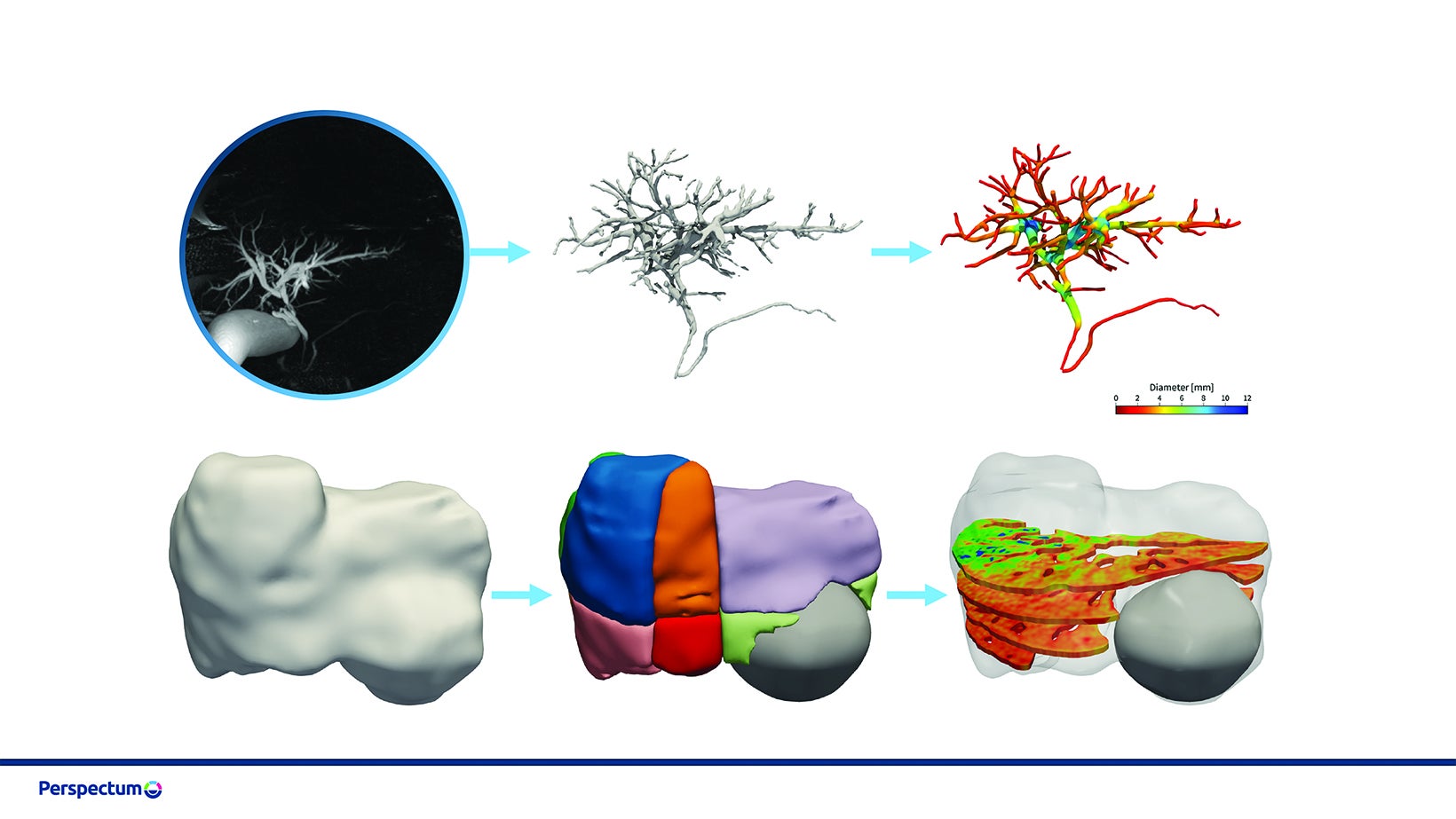
The growing prevalence of liver disease is driving unprecedented demand for noninvasive approaches to diagnose, stratify, and treat this patient population. Radiology is ideally positioned to meet this demand; however, an increasingly stretched workforce necessitates the development and adoption of technologies that can optimize workflow.
The potential of artificial intelligence (AI) in radiology has been well covered, with no shortage of research articles highlighting its utility across the entire workflow — from image acquisition and reconstruction through diagnosis and reporting. Realizing the full benefits of AI, however, necessitates its adoption in regulatory-cleared products that can integrate seamlessly into the radiology workflow.
Challenges in Liver Disease Management
Although intrinsically linked, liver diseases can be broadly divided into two categories: chronic conditions, such as fatty liver disease or hepatitis C; and malignancies, such as hepatocellular carcinoma (HCC) or secondary liver cancers. Both chronic liver diseases and cancer share the key challenges of detection, stratification, prognostication, treatment planning, and monitoring progression or response.
Imaging Chronic Liver Diseases
Nonalcoholic fatty liver disease (NAFLD) is the most prevalent liver disease. It is tightly coupled with metabolic syndrome and is characterized by excess fat accumulation in the liver due to nonalcoholic causes. More than a quarter of adults in the US have NAFLD, with at least one-fifth of these having the higher risk sub-type, nonalcoholic steatohepatitis (NASH). Identifying patients with NASH is essential given the increased risk of poor outcomes and the development of cirrhosis, cancer, cardiovascular diseases, and other conditions.
Traditionally, biopsy has been used to characterize fat and fibroinflammatory activity in the liver. However, the scale of the population, combined with the invasive nature and complications associated with the procedure, have catalyzed development of noninvasive alternatives such as ultrasound- and magnetic resonance-based elastography, and multiparametric quantitative MRI. These quantitative techniques reduce the subjectivity and inter-reader variability associated with conventional qualitative imaging.
Manually delineating organs is tedious, time consuming, and arguably not an effective use of already time-limited radiologists. Advancements in AI-based approaches to liver segmentation can address this problem by facilitating extraction of image-derived liver health metrics.
For example, convolutional neural network (CNN)-based approaches such as 2D and 3D U-Nets have been widely used to segment liver volumes from CT and MR images. Applications include calculating liver volumetry and hepatic functional reserve from Gd-EOB-DTPA-enhanced MRI, quantification of liver iron from R2 maps with FerriSmart, and evaluating fibro-inflammatory activity and fat burden from iron-corrected T1 (cT1) and proton density fat fraction (PDFF, the concentration of fat in a tissue) maps, respectively, in LiverMultiScan.
Moving beyond the parenchyma, AI is being used to aid in evaluating the biliary tree. For example, MRCP+ uses AI-driven pathfinding and tubular enhancement techniques to construct a quantitative parametric model of the biliary tree and pancreatic ducts from standard magnetic resonance cholangiopancreatography (MRCP) images (Figure 1). Biomarkers derived from this model have been shown to predict future liver failure in patients with biliary disease.
Imaging Cancer in the Liver
Hepatocellular carcinoma is expected to become the third-most common cause of cancer-related death in the US within 10 years. This is driven by a combination of the explosion in metabolic-related conditions such as NAFLD and delayed diagnosis and poor treatment outcomes.
Convolutional neural networks have been shown to detect HCC on MRI scans with performance comparable to junior radiologists, although expert radiologists currently remain superior. As with characterizing parenchymal tissue, CNNs have been used to integrate clinical data and blood-based biomarkers with imaging to improve performance of imaging biomarkers. Approaches like this are being evaluated in large-scale clinical studies such as the ELEGANCE trial in Singapore and the DeLIVER study in the United Kingdom.
Both CNN-based approaches and a combination of radiomics with more traditional machine learning methods (eg, support vector machines) have been used to help classify lesions into LI-RADS stages. Clinical adoption of AI techniques to detect and classify lesions can potentially identify cancer at earlier stages and help standardize reporting. Standardization will also accelerate development and translation of new AI technologies.
Tissue segmentation and characterization also play an important role in treatment planning for liver cancer; some AI-based methods are already available in the clinic. GE’s Liver ASSIST Virtual Parenchyma supports liver embolization planning by simulating injections in real time from cone beam CT. The MeVis Liver Suite provides detailed segmentations of the liver, vasculature, and lesions from MR or CT images using CNNs in combination with conventional classifiers. These can be used to inform surgical planning and catheter-based therapies such as transarterial chemoembolization (TACE), in which chemotherapy is delivered locally to the tumor followed by embolization of those vessels. Hepatica combines 3D, U-Net-based segmentation with multiparametric MRI-based biomarkers of fibro-inflammation (cT1) and fat (PDFF) to evaluate the health of the future liver remnant and help inform surgical risk (Figure 2).
Bridging the gap to the clinic
All these opportunities for AI in liver imaging reflect those seen in other indications. Widespread adoption, however, will require incorporating these tools into regulatory-cleared medical devices, which in turn requires robust and well-validated algorithms across different scanner systems and patient populations. They must also fit seamlessly into radiology workflows without requiring switching between multiple software applications, which has previously hampered the use of AI-based segmentation algorithms.
Products such as LiverMultiScan, MeVis Liver Suite, and MRCP+ address this “software-switching” issue by adopting a software-as-a-service (SaaS) approach, whereby AI-based processing takes place remotely, results are delivered automatically and securely to the facility’s picture archiving and communication systems. The importance of SaaS to improve access and outcomes while reducing costs has been recognized in a recent proposal from the US Centers for Medicare and Medicaid Services and the US Department of Health and Human Services to increase reimbursements for technologies such as Heartflow-FFR, LiverMultiScan, and MRCP+.
New treatments for chronic liver diseases, such as NAFLD, along with innovation in cancer care, means radiology’s role in managing these conditions will continue to grow, especially with the help of AI. These are indeed exciting times for liver imaging.
Mr Kelly is the chief innovation officer for Perspectum, based in Oxford, England.