DL-Based Model Predicts Mortality From Pneumonia Using Chest Radiographs
Images
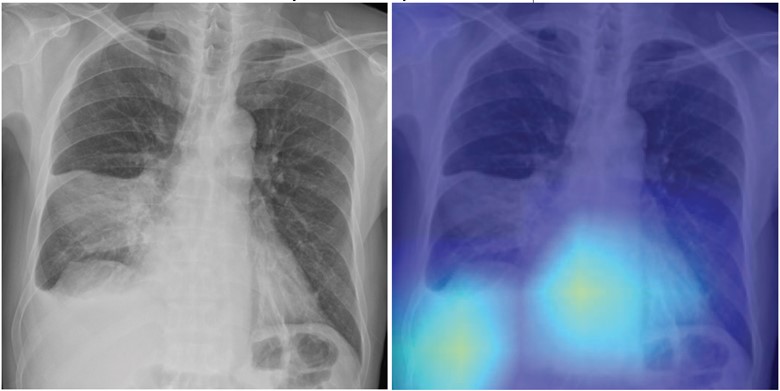
A deep learning (DL)-based model using initial chest radiographs predicted 30-day mortality in patients with community-acquired pneumonia (CAP), improving upon the performance of an established risk prediction tool (i.e., CURB-65 score), according to a study published in the American Journal of Roentgenology.
“The deep learning model may guide clinical decision-making in the management of patients with CAP by identifying high-risk patients who warrant hospitalization and intensive treatment,” concluded first author Eui Jin Hwang, MD, PhD, from the department of radiology at Seoul National University College of Medicine in Korea.
In this AJR accepted manuscript, a DL model was developed in 7,105 patients via one institution from March 2013 to December 2019 (3:1:1 allocation to training, validation, and internal test sets) to predict risk of all-cause mortality within 30 days after CAP diagnosis using patients’ initial chest radiograph. Hwang et al. then evaluated their DL model in patients diagnosed with CAP during emergency department visits at the same institution as the development cohort from January 2020 to December 2020 [temporal test cohort (n = 947)], and from two additional different institutions [external test cohort A (n = 467), January 2020 to December 2020; external test cohort B (n = 381), March 2019 to October 2021]. AUCs were compared between the DL model and a risk score based on confusion, blood urea nitrogen level, respiratory rate, blood pressure, and age ≥ 65 years.
Ultimately, a DL model using initial chest radiographs predicted 30-day all-cause mortality in patients with CAP with AUC ranging from 0.77 to 0.80 in test cohorts from different institutions. Additionally, the model showed higher specificity (range, 61–69%) than the CURB-65 score (44–58%) at the same sensitivity (all p < .001).