Deep-Learning Improves Diagnosis, Management of Soft Tissue Sarcomas
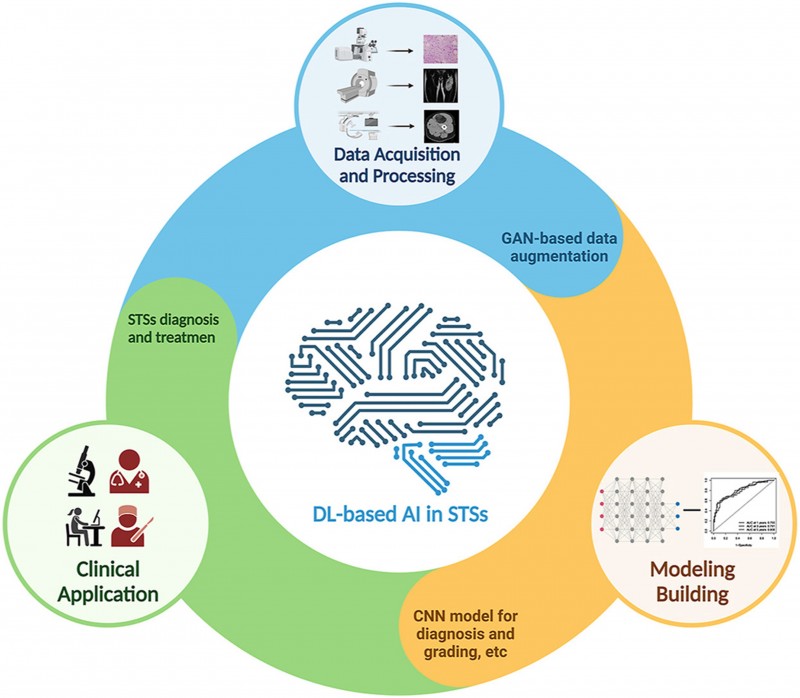
Soft tissue sarcomas (STSs) represent a diverse group of tumors that pose significant diagnostic and therapeutic challenges. In a recent review published in the KeAi journal Meta-Radiology, a team of researchers from The Second Xiangya Hospital at Central South University in Changsha, China, explored the potential application of deep learning (DL) in revolutionizing the management of these complex tumors.
"Deep learning has shown remarkable promise in various medical fields, and its application in STSs is no exception. Our review has synthesized the most recent advancements and highlighted how deep learning can improve the accuracy of diagnosis, personalize treatment plans, and predict patient outcomes more effectively and efficiently,” shares Zhihong Li, senior and co-corresponding author of the study.
The review covers several key areas where deep learning is making an impact:
- Data Acquisition and Processing: The integration of multi-modal data, including radiographic images and histopathological slides, enhances the diagnostic process.
- Algorithm Development: Advanced deep learning models such as convolutional neural networks (CNNs) and generative adversarial networks (GANs) have been developed to improve image analysis and data augmentation.
- Clinical Applications: Deep learning models have been successfully used to automate the contouring of gross tumor volumes (GTVs) for radiation therapy, predict treatment responses, and stratify patients based on risk.
- Pathological Diagnosis: Automation of diagnostic systems using deep learning algorithms can assist pathologists in accurately classifying STS subtypes and identifying prognostic biomarkers.
Prof. Chao Tu, who led the study alongside Li, emphasized the importance of high-quality data and optimized algorithms. "The success of deep learning in clinical applications depends heavily on the quality of the input data and the robustness of the algorithms. Our review has underscored the need for well-annotated datasets and continuous algorithm refinement."