AI in Radiology: A Progress Report
Images
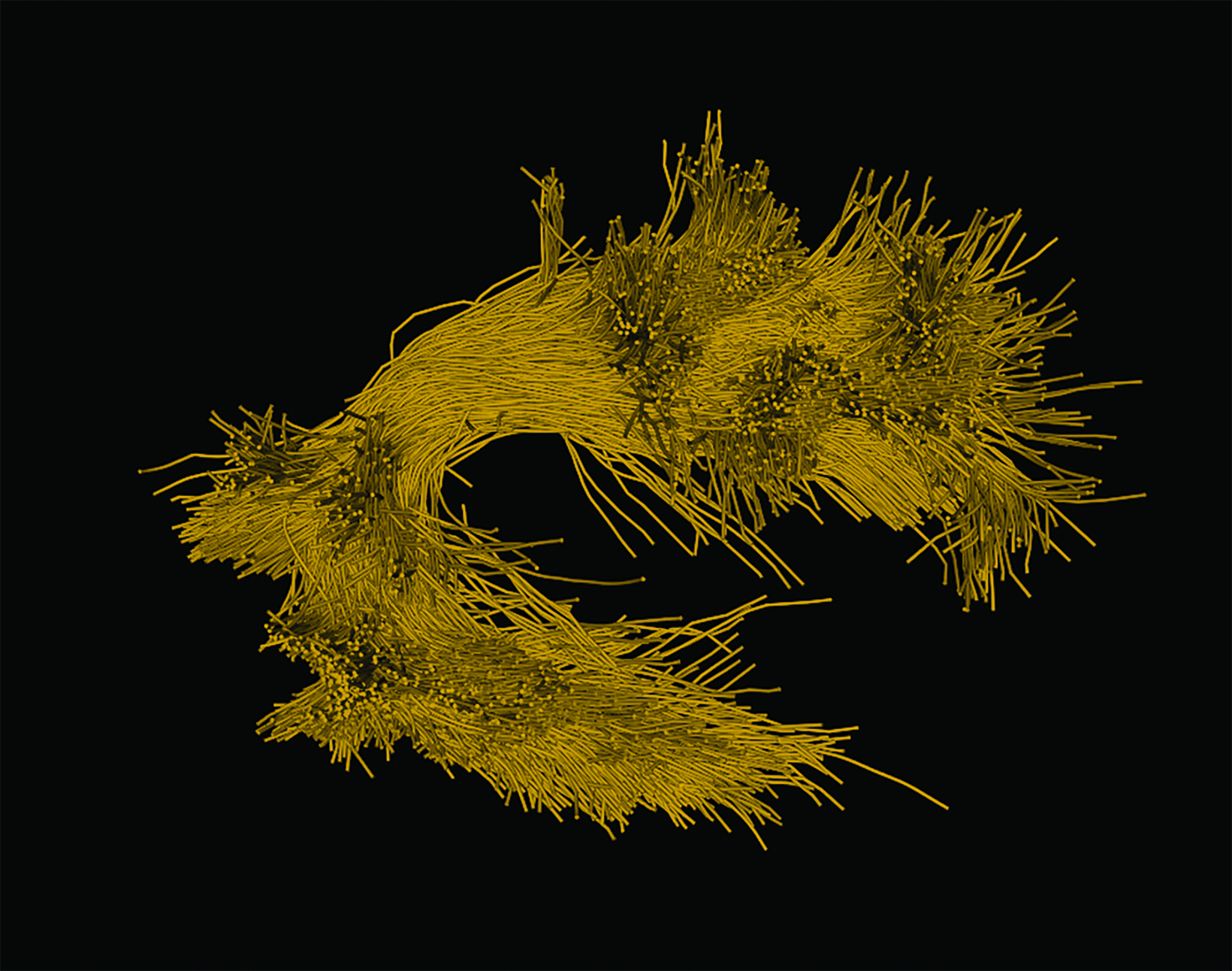
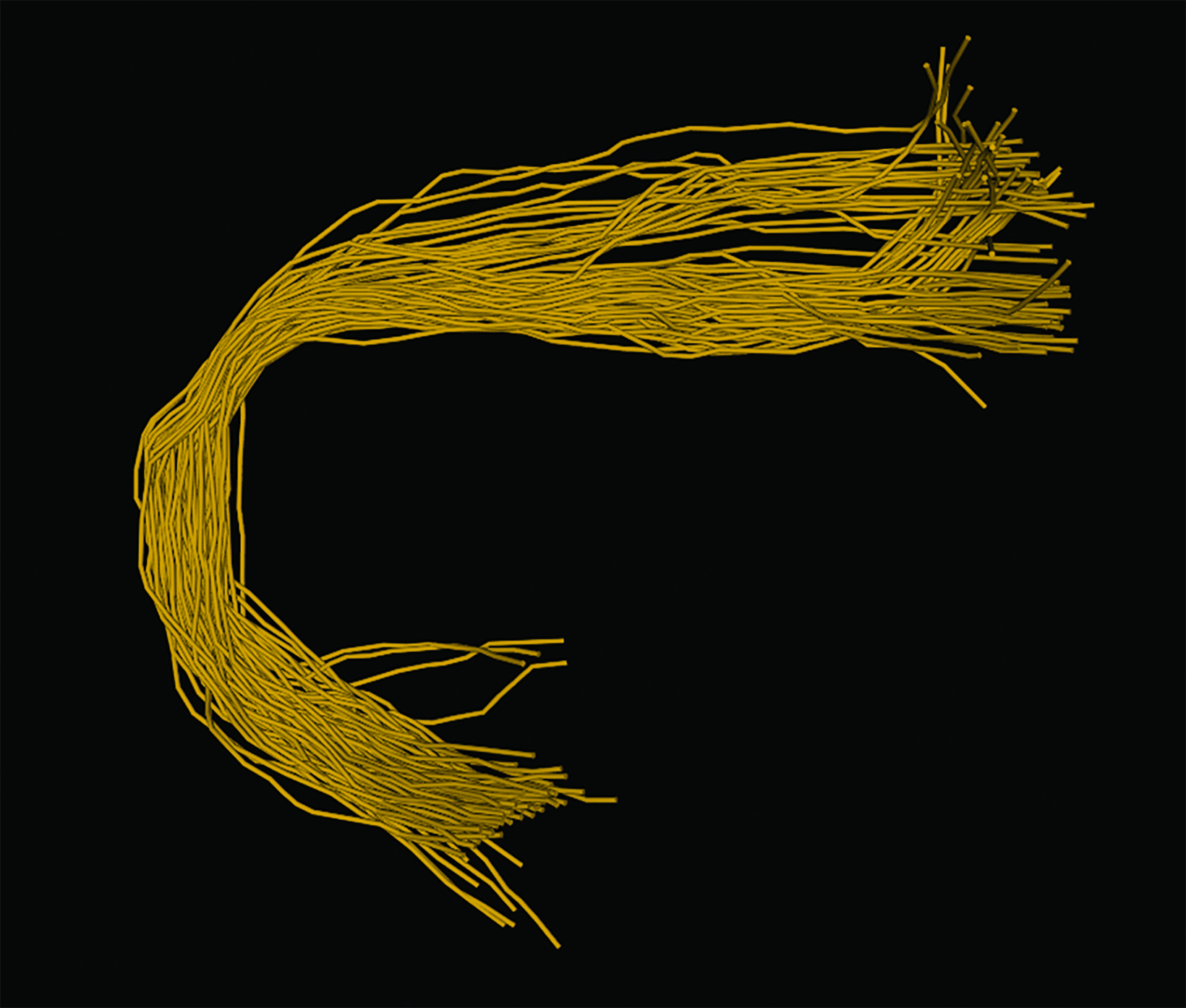
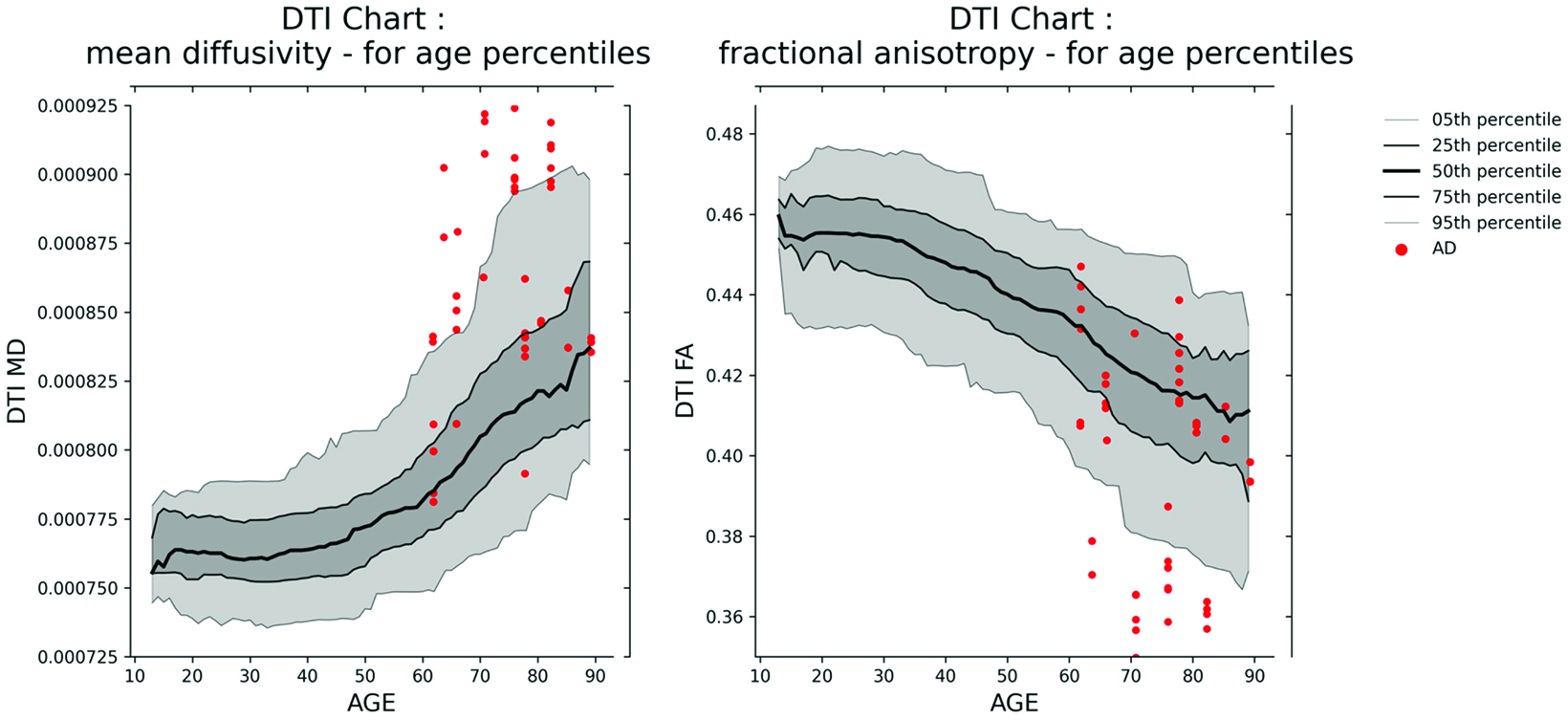
AI has made great strides in recent years in demonstrating numerous benefits for researchers, medical administrators, physicians, and patients. So much so that machine learning (ML) is often seen as the bedrock of tomorrow’s medicine.
As of today, the most commonly targeted applications for medical ML are image and signal processing, disease diagnosis and prognosis, drug discovery, large medical databases, electronic health record (EHR) management, innovative treatments, computer- assisted medical interventions, and gene manipulation. In addition, many medical generative models, all to some degree inspired by chatGPT, have given rise to surprisingly accurate medical chatbots.
However, these advances have been more the prerogative of academic researchers than of clinicians and their patients, as few AI-powered software solutions have made their way to the market. Last year, the FDA reported that fewer than 200 AI/ML medical devices have received premarket clearances, an arguably low number considering the market size and the capabilities of large neural networks.1
There are several reasons for this. First, getting AI/ML software approved by regulatory bodies such as the FDA is difficult. These agencies impose so-called Good Machine Learning Practices that go far beyond the standards commonly used by the research community.2 The FDA also imposes principles of transparency and explainability, whereby the process by which an AI solution makes a decision must be understood by a physician, something often incompatible with the “black box” nature of deep neural networks.
In addition, despite their power, many intelligent algorithms are poorly integrated into the medical routines of clinicians; this makes it difficult for the medical community to adopt them. What’s more, like any new technology, AI-powered software sometimes struggles to gain the trust and buy-in of physicians and patients.
As of today, 76% of all cleared AI/ML medical devices have an intended use in radiology.1 This means that today’s devices are used primarily for the processing and analysis of medical images, predominantly in MRI, CT, radiography, and ultrasound. In addition, they are applied mostly in the fields of oncology, neuroimaging, pulmonology, cardiology, urology, vascular medicine, and ophthalmology, where their central focus is on volumetry. For example, some devices localize, segment, and characterize tumors, while others segment the spatiotemporal dynamics of the ventricles, atria, and myocardium of the heart.
In neuroimaging, many AI/ML medical devices segment and measure regions of the brain like the ventricles, white matter, and gray matter, as well as subregions and deep nuclei such as the hippocampus. Some high-end devices are even used to process and organize white matter fibers extracted from diffusion MRI into meaningful bundles connecting various regions of the gray matter. Such tools (including diffusion tensor imaging, (DTI) have recently been cleared by the FDA.
Soon, the influence of AI will surpass mere volumetric analysis and embed itself within crucial areas of clinical practice. Two such areas are computational processes and diagnostic support. Taking the example of brain DTI analysis, the computational pipelines that support these results involve dozens of sometimes lengthy and error-prone steps. By replacing them with AI, medical devices will become faster and more accurate (Figure 1).2,3
Furthermore, AI is expected to play a central role in the harmonization of data acquired from disparate scanning locales—a notably salient issue with MRI. It will assist in the construction of normative datasets, amalgamating substantial cohorts of normative controls, and highlight the degree of deviation of a patient from this collective. This will permit the evaluation of a patient’s white matter integrity via juxtaposition of DTI metrics pertinent to cerebral microstructure with a reference model.
This will also come with great benefits, since metrics such as fractional anisotropy and mean diffusivity, as well as advanced intra- and extracellular information such as apparent fiber density and free water metrics, have been shown for decades to correlate with assorted neurodegenerative diseases, including Alzheimer, multiple sclerosis, and Parkinson, as well as traumatic brain injury. Figure 2 provides an example of two harmonized DTI normative charts.
While AI is poised to profoundly impact medical practice, many experts suggest it will not supplant human practitioners.4 Instead, AI is envisioned to enhance the daily operations of medical professionals by furnishing them with expeditious, reproducible, and interpretable data analysis software, integrally embedded within the core of their practice.
Citation
Jodoin P. AI in Radiology: A Progress Report. Appl Radiol. 2023;(6):28-29.
November 3, 2023